Luận án Bài toán điều khiển đối với một số lớp hệ tuyến tính dương
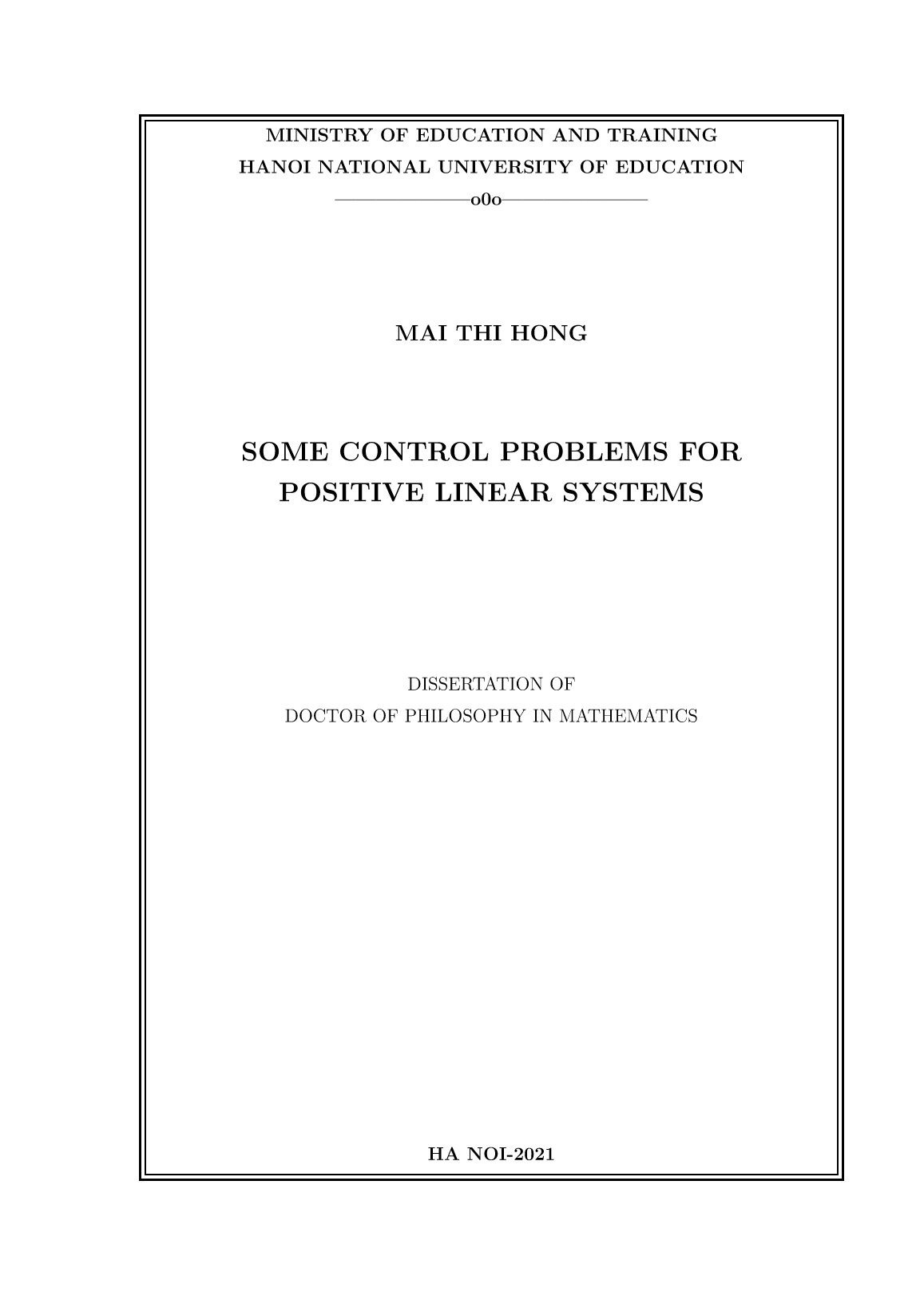
Trang 1
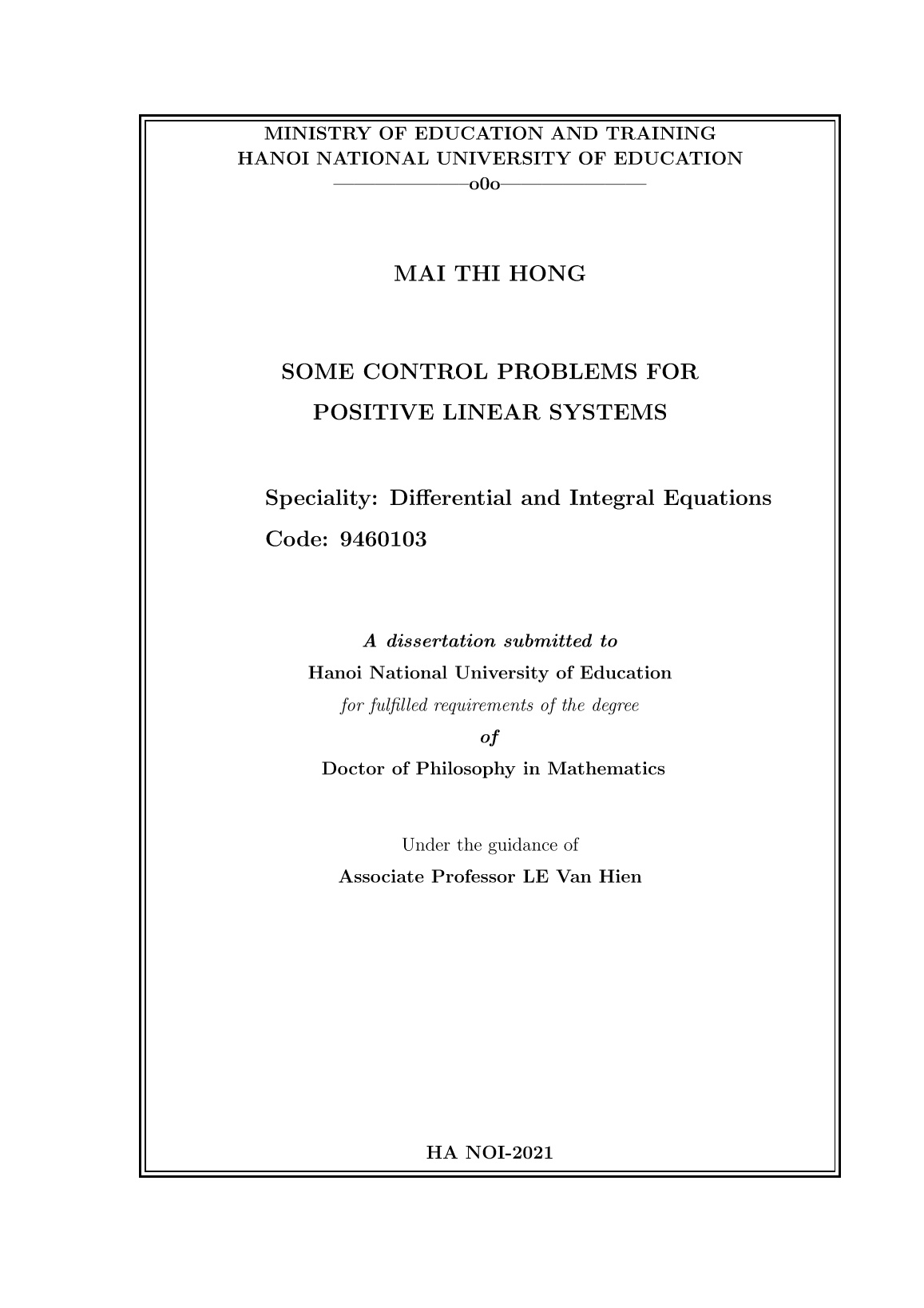
Trang 2
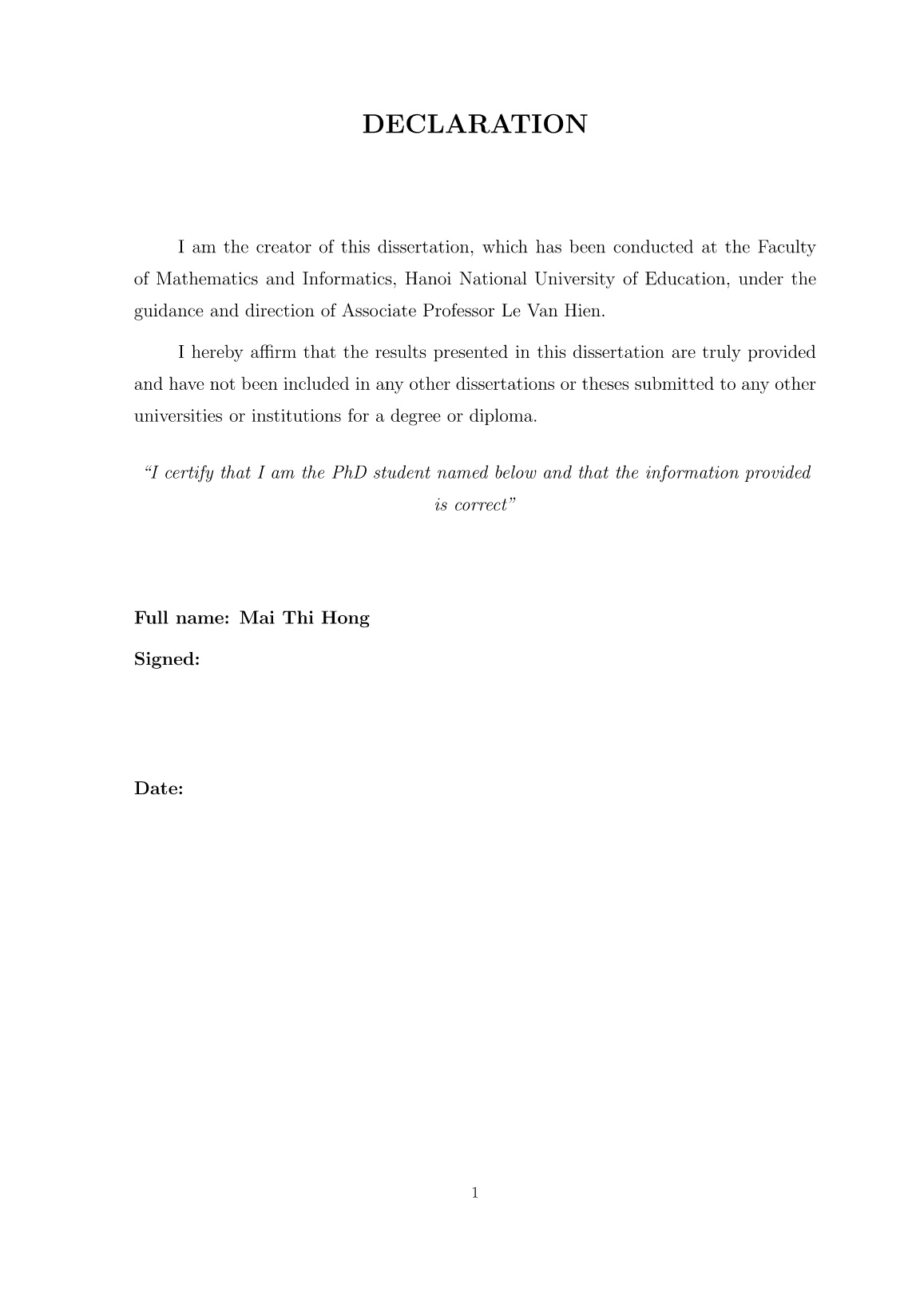
Trang 3
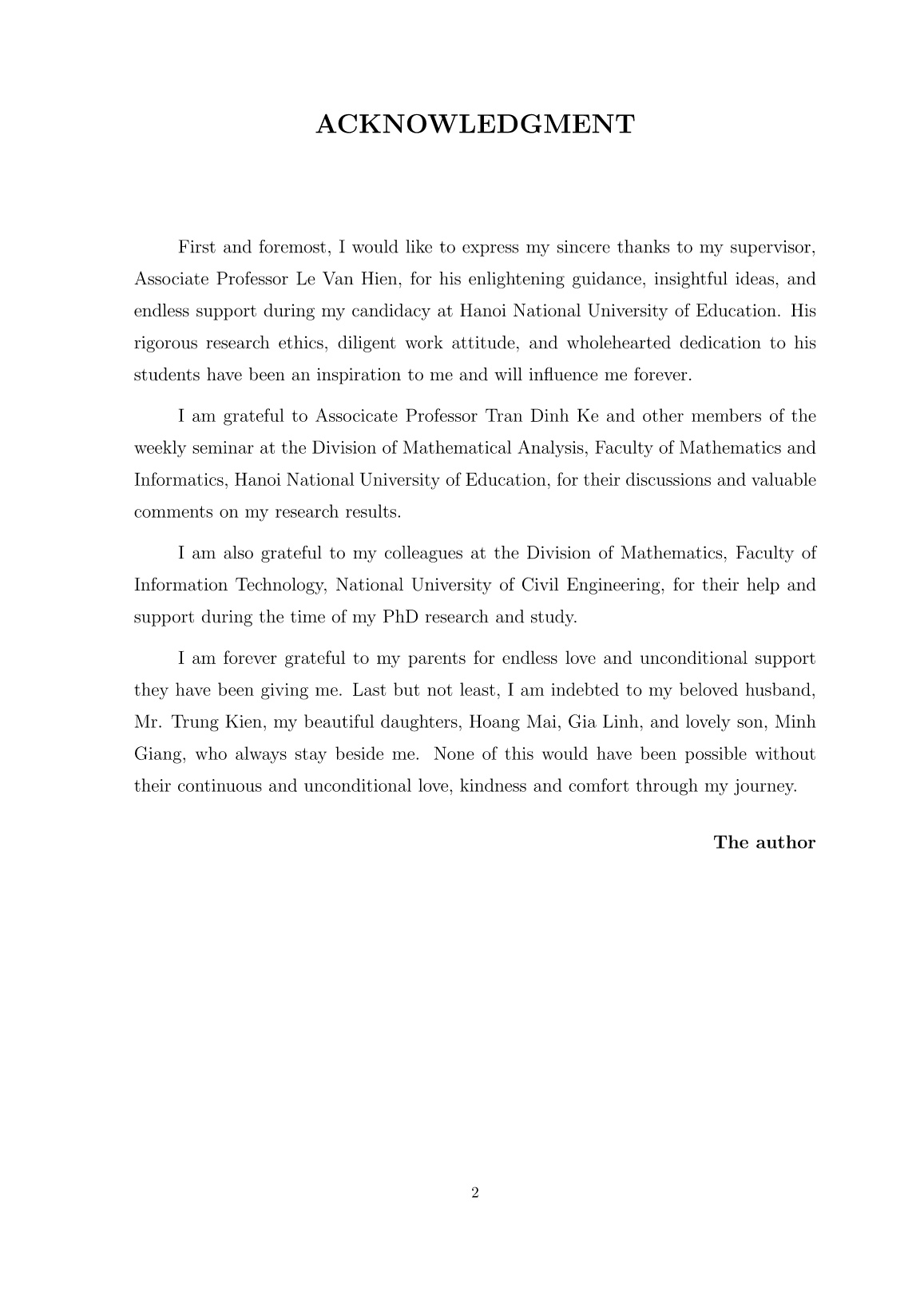
Trang 4
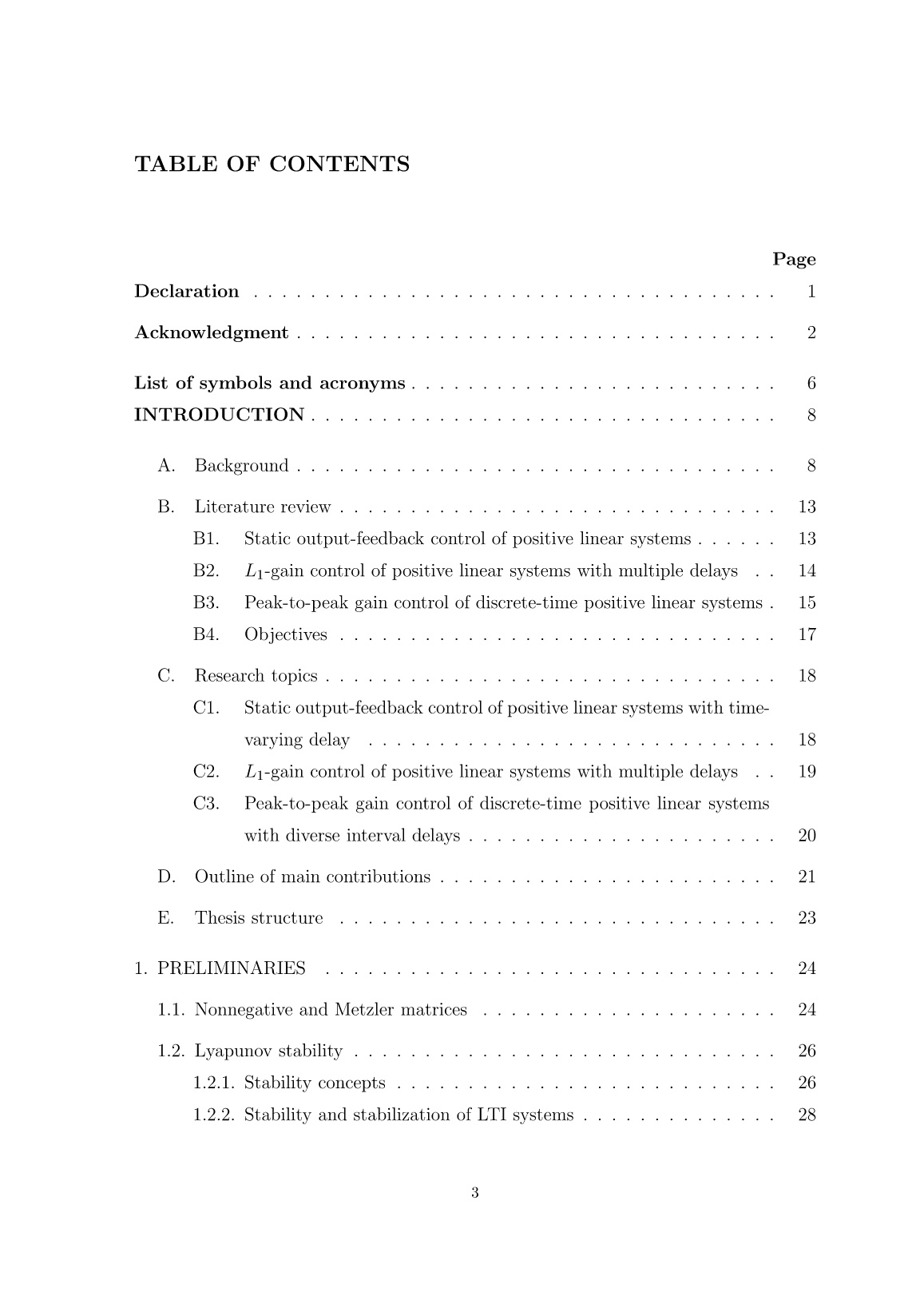
Trang 5
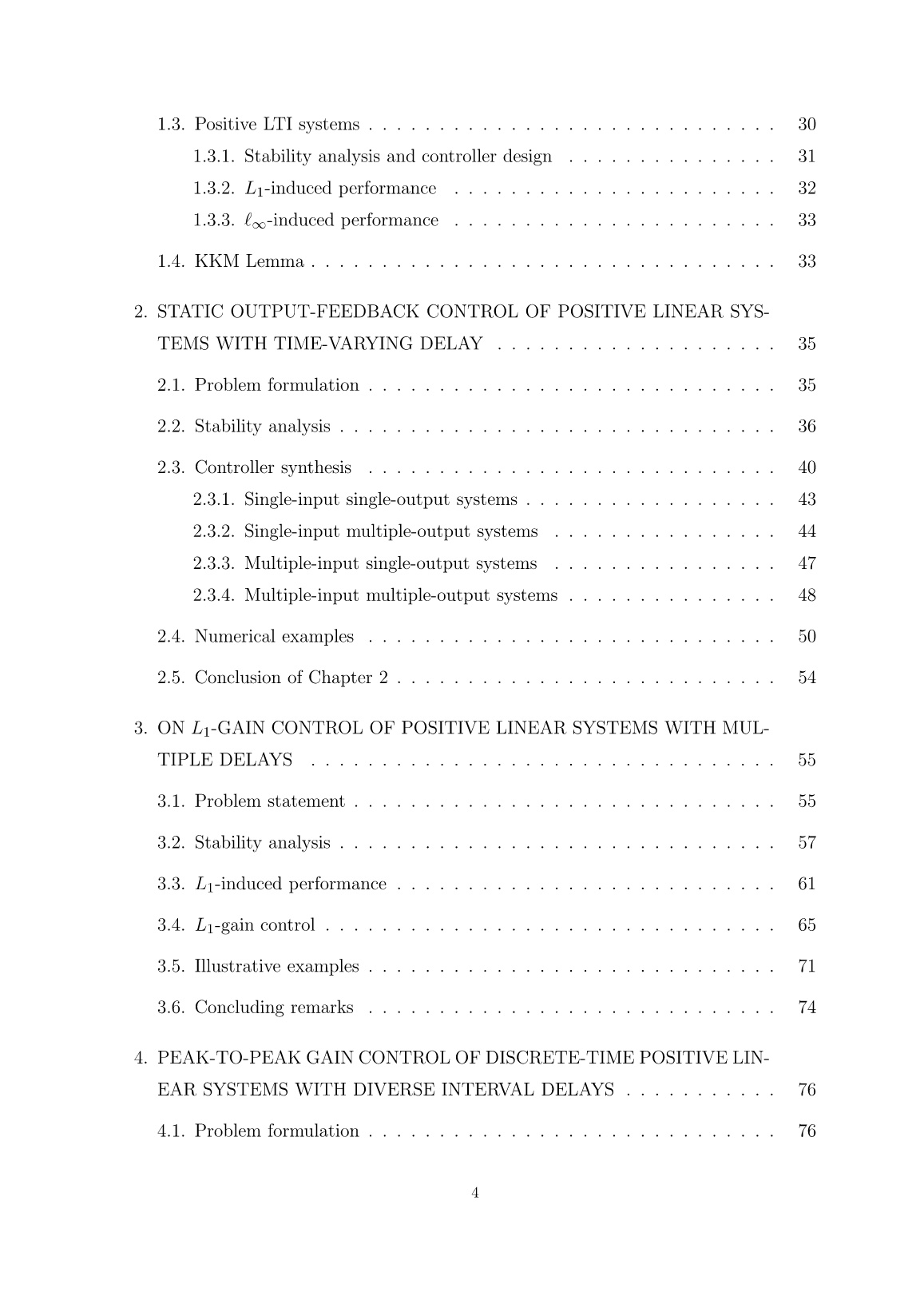
Trang 6
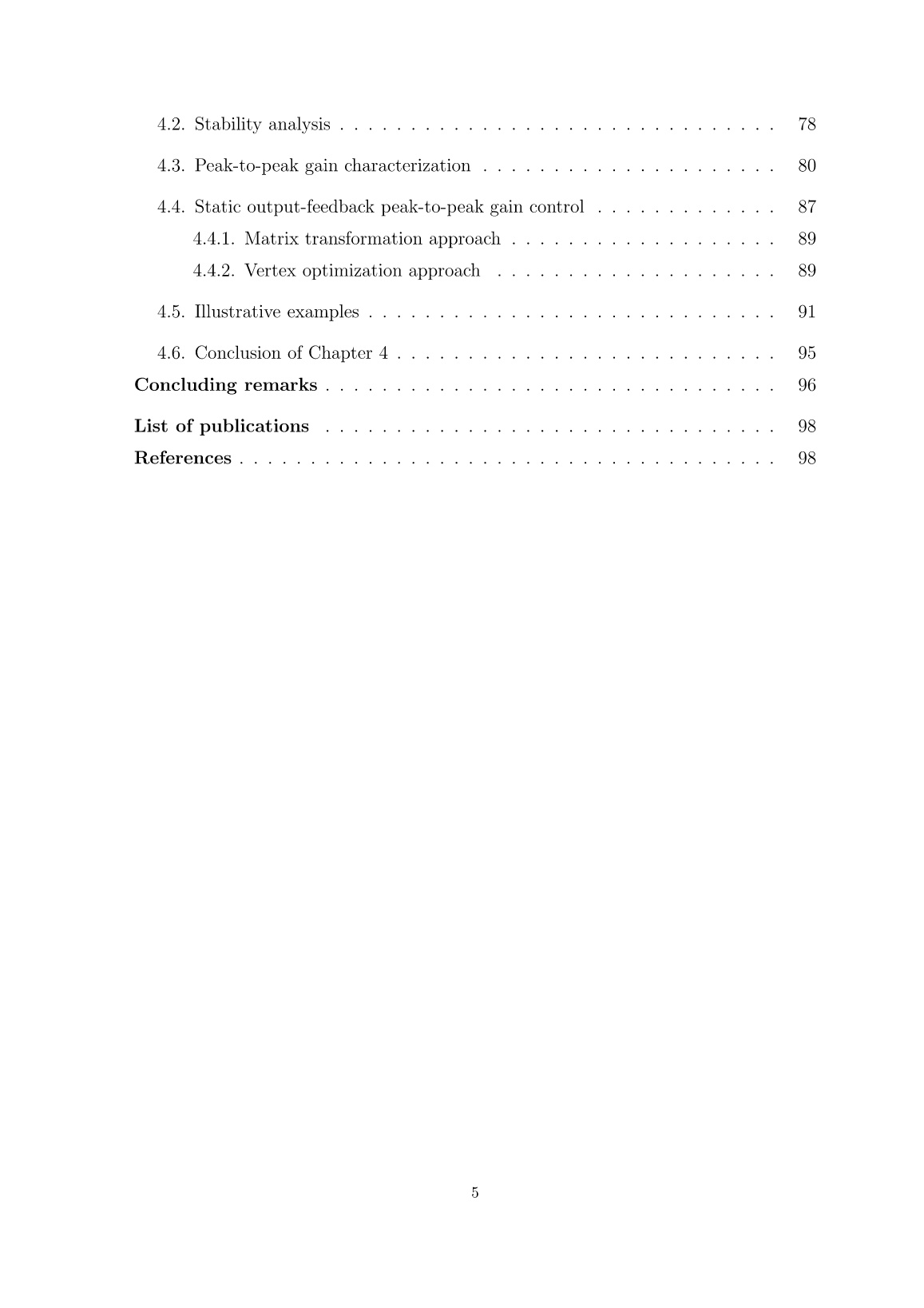
Trang 7
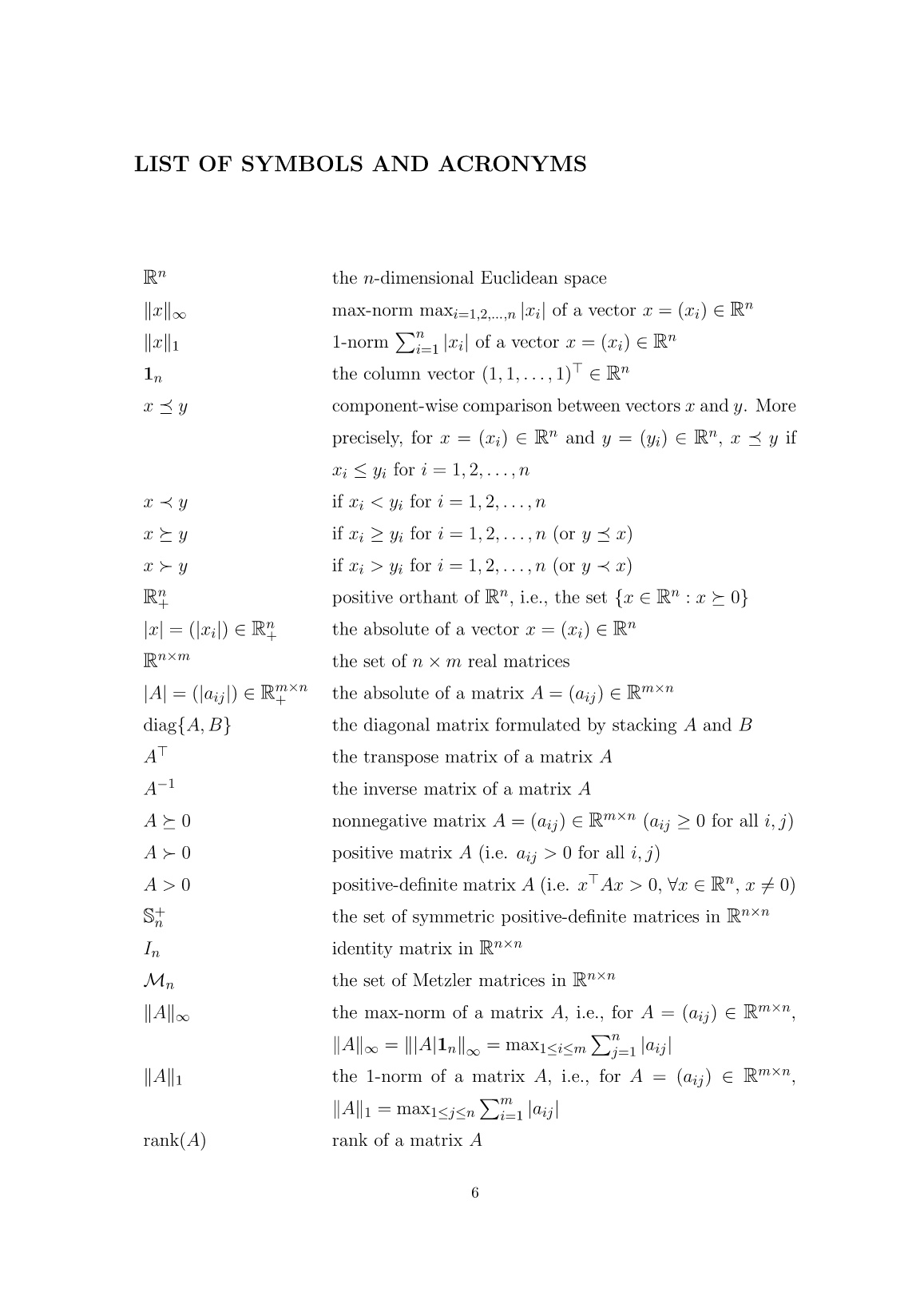
Trang 8
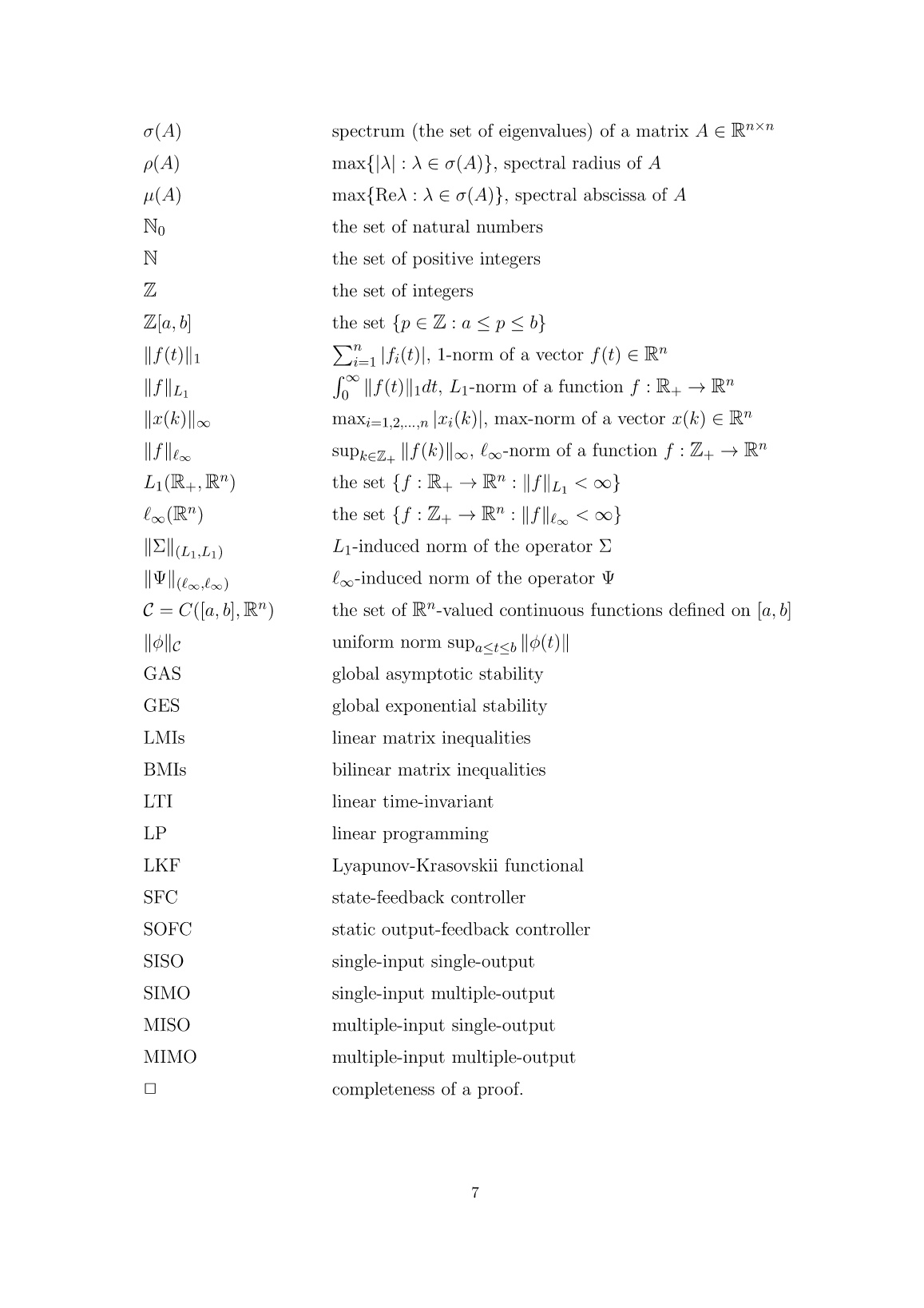
Trang 9
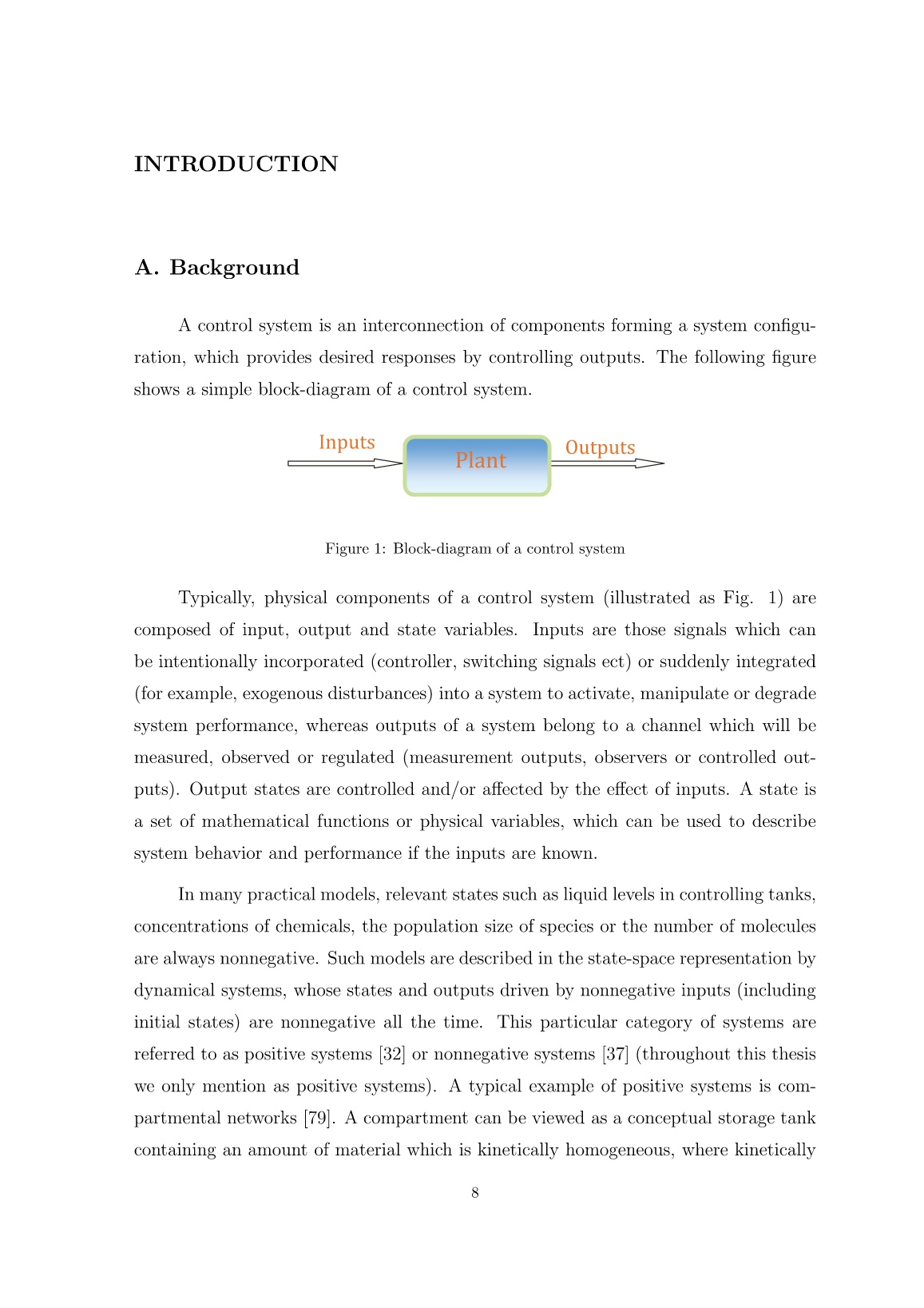
Trang 10
Tải về để xem bản đầy đủ
Bạn đang xem 10 trang mẫu của tài liệu "Luận án Bài toán điều khiển đối với một số lớp hệ tuyến tính dương", để tải tài liệu gốc về máy hãy click vào nút Download ở trên.
Tóm tắt nội dung tài liệu: Luận án Bài toán điều khiển đối với một số lớp hệ tuyến tính dương
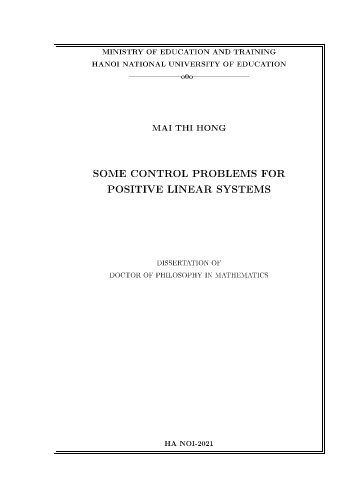
y k = γ ∗ d. (ii) Let γ∗a = mini≠j { aij bicj : bicj ≠ 0 } . Then, the stabilization problem of (2.1) is solvable if and only if the matrix As−γ∗BCs is Hurwitz, where γ∗ = min{γ∗a, γ ∗ d}. In this case, the optimal controller gain is obtained as k = γ∗. Proof. In case (i), the first condition in (2.13) is dropped and the second one holds if and only if k ≤ γ∗d . Then, we have kν ⊤BCs ≼ γ∗dν ⊤ (biciEi +BCd). Thus, the feasibility of condition (2.10c) implies that of condition (2.15). The inverse is also true, where k = γ∗d . Item (ii) can be proved by similar arguments. Remark 2.3.4. Regarding item (i) of Proposition 2.3.2, if Cd = 0 then no threshold of k is imposed. Therefore, when bici ≠ 0, there exists a gain k such that As − kbiciEi is Hurwitz if and only if the submatrix Ais, obtained from As by removing ith row and ith column, is stable. However, when Cd ≠ 0 this condition is no longer applicable, that is, the matrix Ais is stable but the stabilization problem may has no feasible solution. 43 This reflects the effect of time-delay on the system responses. A counterexample is provided in the next section to demonstrate for this assertion, where the stabilization problem of system (2.1) without delayed measurements is always solvable whereas the same problem of (2.1) with delayed measurement output has no feasible solution. See Example 2.4.1 for more details. 2.3.2. Single-input multiple-output systems We now turn our attention to SIMO systems in the form of (2.1). In this case, the controller gain K = k⊤ is a row-vector of dimension p (i.e. k ∈ Rp). We first assume that B = (bi) is not unity (i.e. at least two entries of B are nonzero). Similar to Proposition 2.3.2, we define the constants δ∗ja = min i≠j { aij bi : bi ≠ 0 } , δ∗jd = min i∈1,n { adij bi : bi ≠ 0 } , j ∈ 1, n, and vectors δˆ∗a = [ δ∗1a δ ∗ 2a · · · δ ∗ na ] , δˆ∗d = [ δ∗1d δ ∗ 2d · · · δ ∗ nd ] . Then, condition (2.13) holds if and only if k⊤ [ C Cd ] ≼ [ δˆ∗a δˆ ∗ d ] . (2.16) Revealed by condition (2.16), we define a polyhedron ∆ as follows ∆ = { k ∈ Rp ∣∣ k⊤[C Cd] ≼ [δˆ∗a δˆ∗d]} . (2.17) By the fact rank(C + Cd) ≤ rank[C Cd], it is easy to verify that (2.17) defines a nonempty polyhedron. Let S be the set of vertices of ∆. For a fixed positive vector χ ∈ Rn, we define the function ϱχ(k) = k⊤Csχ and consider the following optimization problem (P ) : maximize {ϱχ(k) : k ∈ ∆} . (2.18) It is obvious that ϱχ(k) = k⊤Csχ ≥ 0 for any efficient solution of (2.18). Let I+ and I− be the sets of indices defined as I+ = { j : k⊤(cj + cdj) ≥ 0 } , I− = { j : k⊤(cj + cdj) < 0 } . Then, by (2.17), we have∑ j∈I− k⊤(cj + cdj)χj ≥ − ∑ j∈I+ k⊤(cj + cdj)χj 44 ≥ − ∑ j∈I+ (δ∗ja + δ ∗ jd)χj , which gives k⊤Cs1n ≥ −µ, where µ = (maxχj)/(minχj)(δˆ∗a + δˆ ∗ d)1n. Thus, the problem (P) is equivalent to maximize { ϱχ(k) : k ∈ ∆˜ } , where ∆˜ = {k ∈ ∆ : k⊤Cs1n ≥ −µ}. Since ϱχ(k) is a continuous function on a compact set ∆˜, the solution set Sol(P ) of (2.18) is nonempty. Moreover, the following result indicates that Sol(P ) can be taken among vertices in S. Lemma 2.3.1. For any k∗ ∈ Sol(P ), there exists a vertex kv ∈ S such that ϱχ(kv) = ϱχ(k∗). Proof. Let k∗ ∈ Sol(P ). If k∗ is an interior point of ∆ then, for sufficiently small ϵ > 0, kϵ = k∗ + ϵ1p ∈ ∆. Thus, ϱχ(kϵ) = k ⊤ ∗ Csχ+ ϵ∥Csχ∥1 > ϱχ(k∗). This contradicts with ϱχ(k∗) is the maximum of ϱχ(k) on ∆. Note also that k∗ does not fall within the hyperplane k⊤Cs1n = −µ. Thus, k∗ must be within the boundary of ∆ and, consequently, we can represent as k∗ = ∑s j=1 λjkj, where kj ∈ S and λj ≥ 0,∑s j=1 λj = 1. Note in addition that (k∗ − kj) ⊤Csχ = ϱχ(k∗)− ϱχ(kj) ≥ 0. Therefore, 0 ≤ s∑ j=1 λj(k∗ − kj) ⊤Csχ = ( k∗ − s∑ j=1 λjkj )⊤ Csχ = 0 which gives ϱχ(k∗) = ϱχ(kv) for some kv = kj . We are now in a positive to state our main result in this section. Theorem 2.3.1. Let Assumption (A) hold. The stabilization problem of positive sys- tem (2.1) is solvable if and only if there exists a vertex kv ∈ S such that the matrix As − Bk⊤v Cs is Hurwitz. Equivalently, there exists a vector η ∈ R n, η ≻ 0, satisfying the following LP condition Asη − B ( k⊤v Csη ) ≺ 0. (2.19) Proof. (Necessity) Let k ∈ Rp be a desired controller gain. Then, k satisfies condition (2.16) and the matrix As − Bk⊤Cs is Hurwitz. Consequently, there exists a vector 45 η ∈ Rn, η ≻ 0, such that ( Bk⊤Cs − As ) η ≻ 0. If k /∈ S then we set the problem (2.18) with χ = η. By Lemma 2.3.1, there exists a vertex kv ∈ S such that k⊤v Csη = max{k ⊤Csη : k ∈ ∆}. Clearly, Bk⊤v Csη ≽ Bk ⊤Csη. Therefore, ( Bk⊤v Cs −As ) η ≻ 0. This shows that the matrix As−Bk⊤v Cs is Hurwitz. Sufficient part is obvious. The proof is completed. Remark 2.3.5. Typically, the vertex kv in the statement of Theorem 2.3.1 satisfies some maximal properties. By this observation, kv can be found as a vertex that maximizes that objective function ϱ1n(k) = k ⊤Cs1n. Remark 2.3.6. When B is unity, that is, B has only one nonzero entry namely bj , the component δ∗ja of δˆ ∗ a is set to a given upper bound. Then, the result of Theorem 2.3.1 is still valid. Remark 2.3.7. The result of Theorem 2.3.1 can be extended to multiple-input multiple- output (MIMO) systems, where the matrix B has unity rows, that is, each row of B has at most one non-zero entry. Specifically, let us define θa∗lj = min i≠j { aij bil : bil ≠ 0 } , θd∗lj = min i∈1,n { adij bil : bil ≠ 0 } , j ∈ 1, n, l ∈ 1, m, θa∗l = [ θa∗l1 θ a∗ l2 · · · θ a∗ ln ] , θd∗l = [ θd∗l1 θ d∗ l2 · · · θ d∗ ln ] and the polyhedron ∆l is defined as ∆l = { kl ∈ R p ∣∣ k⊤l [C Cd] ≼ [θa∗l θd∗l ]} . (2.20) We have the following result. Proposition 2.3.3. Let Assumption (A) hold and assume that the matrix B has unity rows and each column of B is not unity. Then, the stabilization problem of positive system (2.1) is solvable if and only if there exist vertices kvl of ∆l, l ∈ 1, m, such that the matrix As −BKvCs is Hurwitz. The optimal controller gain matrix is given as Kv = [ kv1 k v 2 · · · k v m ]⊤ . Proof. The proof is similar to that of Theorem 2.3.1 with observations that [Ac]ij = aij − m∑ l=1 bil(k ⊤ l cj) ≥ 0 46 for i ≠ j if and only if k⊤l cj ≤ θ a∗ lj and [Adc]ij = adij − m∑ l=1 bil(k ⊤ l cdj) ≥ 0 if and only if k⊤l cdj ≤ θ d∗ lj . Thus, k ⊤ l [C Cd] ≼ [θ a∗ l θ d∗ l ]. The remaining of the proof is omitted. Remark 2.3.8. When the matrix B has unity rows, the summation ∑m l=1 bil(k ⊤ l cj) is reduced to only one term. Thus, we can handle the synthesis process of each vector kl without coupling of B and C. This feature simplifies the derivation of Proposition 2.3.3. However, for general MIMO systems, a similar separation is no longer available. In the remaining of this section, complete solutions to the stabilization problem of positive systems in the form of (2.1) are given for two cases of multiple-input single- output (MISO) systems and MIMO systems with purely delayed measurements. 2.3.3. Multiple-input single-output systems Let us consider a class of MISO systems given in (2.1). In this case, the controller gain K = (kl) ∈ R m is a column-matrix. Let aˆi = min i≠j { aij cj : cj ≠ 0 } , aˆdi = min j∈1,n { adij cdj : cdj ≠ 0 } , a∗i = min{aˆi, aˆdi}, i ∈ 1, n, where the min operation on empty set will be ignored. Then, condition (2.13) holds if and only if k belongs to the polyhedron ∆ˆ defined as ∆ˆ = { x ∈ Rm : m∑ l=1 bilxl ≤ a ∗ i , i ∈ 1, n } . (2.21) Let Sˆ be the set of vertices of ∆ˆ. We have the following result. Proposition 2.3.4. Let Assumption (A) hold. The stabilization problem of MISO pos- itive systems in the form of (2.1) is solvable if and only if there exists a vertex kv ∈ Sˆ such that the matrix As−BkvCs is Hurwitz. An optimal controller gain, which assures the fastest convergence rate of the closed-loop system, is an efficient point K = k∗v of the vector-valued optimization problem max m∑ l=1 kvl (b ⊤ l Cs) s.t. kv ∈ Sˆ and As − BkvCs is Hurwitz. (2.22) 47 In order to prove the latter statement in Proposition 2.3.4, we recall here the following interesting result stated in [69, Lemma 10]. Lemma 2.3.2. Let A ∈ Rn×n be a Metzler matrix and A+1 , A + 2 ∈ R n×n + be matrices such that A+1 ≼ A + 2 . If A− A + 1 and A− A + 2 are Metzler matrices, then we have λmax(A− A + 1 ) ≥ λmax(A−A + 2 ). Proof. (of Proposition 2.3.4) We notice at first that the stabilization problem of MISO positive system (2.1) is solvable if and only if there exist a vector k ∈ ∆ˆ and a positive vector ν ∈ Rn satisfying the following condition ν⊤As − m∑ l=1 kl ( b⊤l ν ) Cs ≺ 0. (2.23) We denote asj the jth column of As. Then, it follows from (2.23) that σK ! m∑ l=1 (b⊤l ν)kl > ν ∗ a ! max j∈1,n { ν⊤asj cj + cdj : cj + cdj ≠ 0 } . (2.24) If k is not a vertex of ∆ˆ then we can set the LP problem maximize σK subject to K ∈ ∆ˆ. By Lemma 2.3.1, there exists a vertex kv such that σkv = maxK∈∆ˆ σK . According to (2.24), it is clear that (2.23) is also satisfied with k = kv. Thus, the matrix As−BkvCs is Hurwitz. Let kv ∈ Sˆ be a vertex of ∆ˆ such that As − BkvCs is Hurwitz. Then, αkv = −λmax(As − BkvCs) is a positive constant that determines the convergent rate of the closed-loop system. By Lemma 2.3.2, to maximize the convergent rate αkv , the vertex kv will be selected as an efficient point of the problem (2.22). The proof is now completed. 2.3.4. Multiple-input multiple-output systems In this section, we address the stabilization problem for MIMO systems given in (2.1) with purely delayed measurements, that is, C = 0. By Assumption (A), Cd has full-row rank (i.e. rank(Cd) = p). We assume that Cd has a particular row 48 echelon form [65] as [C¯d 0p×(n−p)], that is, there exists a nonsingular matrix L such that LCd = [C¯d 0p×(n−p)], where C¯d ∈ R p×p has full-rank. By using the change of variable K = K¯C¯−1d L, (2.25) condition (2.13) holds if and only if BK¯ ≼ A¯d, where A¯d is the submatrix composed of the first p columns of Ad. Thus, each column k¯j of K¯ belongs to the following polyhedron ∆j = { k¯j ∈ R m ∣∣∣ m∑ l=1 bilk¯lj ≤ adij , i ∈ 1, n } Let Sj be the set of vertices of ∆j , j ∈ 1, p. Our main result in this section is presented in the following theorem. Theorem 2.3.2. Consider positive system (2.1) with C = 0 and Cd has its row echelon form [C¯d 0p×(n−p)]. Then, under Assumption (A), the stabilization problem of (2.1) is solvable if and only if there exists a set of vertices k¯vj ∈ Sj, j ∈ 1, p, such that the matrix As − B[K¯v 0p×(n−p)] is Hurwitz. An optimal controller gain is given as K∗ = K¯∗v C¯ −1 d L, where K¯ ∗ v = [ k¯∗1 k¯ ∗ 2 · · · k¯ ∗ p ] and each k¯∗j , j ∈ 1, p, is an efficient point of the problem max m∑ l=1 k¯vljbl s.t. k¯ v j ∈ Sj and As −B[K¯v 0p×(n−p)] is Hurwitz. Proof. LetK be a desired controller gain then, according to (2.25), K¯ = [ k¯1 k¯2 · · · k¯p ] , where k¯j ∈ ∆j , and As−BKCd is Hurwitz. Note also that BKCd = [ BK¯ 0p×(n−p) ] . Thus, there exists a positive vector ν ∈ Rn such that σk¯j ! m∑ l=1 (b⊤l ν)k¯lj > ν ⊤asj , ∀j ∈ 1, p. Similar to Proposition 2.3.4, if k¯j /∈ Sj , let k¯vj ∈ Sj be a vertex that maximizes σk¯j on ∆j . Then, we have σk¯vj ≥ σk¯j > ν ⊤asj . Thus, As −BKvCd is also Hurwitz, where Kv = K¯vC¯ −1 d L and K¯v = [ k¯v1 k¯ v 2 · · · k¯ v p ] . The remaining of the proof is similar to that of Proposition 2.3.4 and we omit here. 49 2.4. Numerical examples In this section, we give some numerical examples to illustrate the effectiveness of the derived stabilization conditions in the preceding section. Example 2.4.1. Consider system (2.1) where A = ⎡⎢⎢⎣ −1 1 1 1 −1 0 1 0 −2 ⎤⎥⎥⎦ , Ad = ⎡⎢⎢⎣ 1 1 1 0 0 1 1 1 0 ⎤⎥⎥⎦ , B = ⎡⎢⎢⎣ 1 0 0 ⎤⎥⎥⎦ = C⊤. Since the matrix As = ⎡⎢⎢⎣ 0 2 2 1 −1 1 2 1 −2 ⎤⎥⎥⎦ is not Hurwitz, the open-loop system is unstable. However, the submatrix A1s = [ −1 1 1 −2 ] is obviously a stable matrix. a) The matrix Ac = A − kBC is Metzler for any k while As − kBC is stable if and only if k > 14. Thus, the stabilization problem of system (2.1) with Cd = 0 is always solvable. b) For Cd = [0 1 1], we have γ ∗ d = 1 (i.e. Ad− kBC is nonnegative if and only if k ≤ 1). In this case, the matrix As − γ∗dBCs = ⎡⎢⎢⎣ −1 1 1 1 −1 1 2 1 −2 ⎤⎥⎥⎦ is unstable. Thus, the stabilization problem of (2.1) is not solvable. Example 2.4.2. Consider system (2.1) where A = [ −1 1.2 0.25 −1 + ω0 ] , Ad = [ ω0 0.8 0.75 1 ] , B = [ 1 1 ] , C⊤ = [ 0 1 ] = C⊤d , where ω0 ≥ 0 is a real parameter. By Proposition 2.1.1, system (2.1) is positive. In addition, the open-loop system is unstable for any ω0 ≥ 0. As defined in Proposition 2.3.2, we have γ∗a = 1.2, γ ∗ d = 0.8, and hence γ∗ = min{γ ∗ a, γ ∗ d} = 0.8. Therefore, As − γ ∗BCs = [ −1 + ω0 0.4 1 ω0 − 1.6 ] . By Proposition 2.3.2, the stabilization problem of system (2.1) is solvable if and only if the matrix As − γ∗BCs is Hurwitz yielding that ω0 ∈ [0, 0.6). State trajectories of the 50 closed-loop system for various ω0 ∈ [0, 0.6) with φ = [1 1]⊤ and δ∗ = 3 are given in Fig. 2.1 (a)-(b). It can be seen on Fig. 2.1 that the convergent time increases quickly in accordance to the increasing of ω0 in [0, 0.6). This illustrates for the critical value of ω0 for the solvability of the stabilization problem. t0 50 100 150 200 x 1 (t) 0 0.2 0.4 0.6 0.8 1 ω0=0.5 ω0=0.525 ω0=0.4 ω0=0.55 ω0=0.59 (a) State x1 t0 50 100 150 200 x 2 (t) 0 0.2 0.4 0.6 0.8 1 ω0=0.4 ω0=0.5 ω0=0.525 ω0=0.55 ω0=0.59 (b) State x2 Figure 2.1: State trajectories of the closed-loop system for various ω0 51 Example 2.4.3. Consider the following system x˙(t) = ⎡⎢⎢⎣ a 1 1 1 b 0 1 1 −1 ⎤⎥⎥⎦ x(t) + ⎡⎢⎢⎣ 0.5 0.6 1 0 0.3 0.5 0.7 0.5 1 ⎤⎥⎥⎦ x(t− δt) + ⎡⎢⎢⎣ 1 0 1 ⎤⎥⎥⎦ u(t), y(t) = [ 0 1 1 1 1 0 ] x(t) + [ 1 0 0 0 1 1 ] x(t− δt), (2.26) where a, b are uncertain parameters. Clearly, system (2.26) is positive. Moreover, the corresponding open-loop system is unstable for any a, b. We now determine whether it can be stabilized via SOFC. From (2.16), we have δˆ∗a = [1 1 1] and δˆ ∗ d = [0.5 0.5 1]. Thus, the polyhedron (2.17) has only one nonzero vertex S = {[0.5 0.5]⊤}. With kv = [0.5 0.5]⊤, the matrix As −Bk⊤v Cs is given as Aa,b = ⎡⎢⎢⎣ a− 0.5 0.1 1 1 b+ 0.3 0.5 0.7 0 −1 ⎤⎥⎥⎦ . By Theorem 2.3.1, the stabilization problem of system (2.26) is solvable if and only if Aa,b is Hurwitz. The latter condition is satisfied if and only if (a, b) ∈ Ωa,b defined as Ωa,b = { (a, b) : s(a, b) ! ab+ 0.3a+ 0.2b− 0.075 > 0, b < −0.3 } which is presented in Fig. 2.2 (a)-(b). For example, when b = −1 system (2.26) can be positively stabilized if a < −0.3925 and cannot be positively stabilized if a ≥ −0.3925. Example 2.4.4. Consider system (2.1) where A = ⎡⎢⎢⎣ −5.5 0 0.5 0.3 −1.8 0 1 0 −1.75 ⎤⎥⎥⎦ , Ad = ⎡⎢⎢⎣ 0.5 0.8 0 9 6 0 2 3 1 ⎤⎥⎥⎦ , B = ⎡⎢⎢⎣ 0 1 3 0 2 1 ⎤⎥⎥⎦ , C = 0, Cd = [ 1 1 0 0 1 0 ] . For this example, the open-loop system is also unstable. In addition, Cd =[ C¯d 02×1 ] , where C¯d = [ 1 1 0 1 ] has full-rank and C¯−1d = [ 1 −1 0 1 ] . Now, we utilize Theorem 2.3.2 to design a stabilizing SOFC. For this, we determine the polyhedra ∆j, 52 -2 -2 0 -3 2 s( a, b) 4 -2 5 b -1 a-10 0 1 (a) s(a, b) -5 -4 -3 -2 -1 -0.3 0b -4 -3 -2 -1 -0.2 0 0.5 a Feasible region a,b -0.3925 (b) Ωa,b Figure 2.2: Feasible region of (a, b) j = 1, 2, and find that the sets of vertices of ∆j are given by S1 = {[ 0.75 0.5 ] , [ 3 −4 ]} , S2 = {[ 1.1 0.8 ] , [ 2 −1 ]} . It can be shown by enumeration that only one set of vertices k¯∗1 = [ 3 −4 ] , k¯∗2 = [ 2 −1 ] 53 can stabilize the system. Specifically, the matrix A = As − B [ K¯∗v 02×1 ] , where K¯∗v = [k¯ ∗ 1 k¯ ∗ 2], is given as A = ⎡⎢⎢⎣ −1 1.8 0.5 0.3 −1.8 0 1 0 −0.75 ⎤⎥⎥⎦ with λ(A) = {−0.0161,−2.3317,−1.2022}. Thus, A is Hurwitz. By Theorem 2.3.2, the stabilization problem of system (2.1) is solvable. The optimal controller gain is obtained as K = K¯∗v C¯ −1 d = [ 3 −1 −4 3 ] . It is noted also that, different from the cases of single-input or single-output, for MIMO systems, the controller gain K cannot be restricted to the set that contains only nonnegative matrices. For instance, in this example, if the polyhedra ∆j are subject to constraints k¯j ≽ 0 then, by enumeration, we find that there does not exist any set of vertices that makes the closed-loop system stable. 2.5. Conclusion of Chapter 2 In this chapter, the problem of static output-feedback control has been investi- gated for LTI positive systems with time-varying delay in the state and output vectors. By a vertex optimization approach, necessary and sufficient conditions for the exis- tence of a desired SOFC have been derived in the form of LP problems, which can be effectively solved by various convex algorithms. The results presented in this chapter extend some existing results in the literature, for example, [7,60,92,95]. Moreover, the proposed method in this chapter can also be adapted to various problems in the control theory of positive systems such as the analysis and synthesis under L1/L∞-gain schemes [11,12], interval observer design [29] or state estimation [8]. Extending the results of this chapter to such problems proves to be an interesting and relevant issue, which will be investigated in the next chapters. 54 Chapter 3 ON L1-GAIN CONTROL OF POSITIVE LINEAR SYSTEMS WITH MULTIPLE DELAYS In this chapter, the problem of L1-gain control is studied for a class of positive linear systems with diverse state and output delays. A necessary and sufficient stabil- ity condition is first quoted via the concepts of exponential stability and L1-stability and a new alternative approach using Laplace transformation is proposed to establish a characterization of L1-induced norm of the input-output operator. The obtained L1-induced norm characterization is then utilized to formulate necessary and sufficient conditions subject to L1-induced performance with prescribed level. Then, based on some vertex optimization techniques, a complete solution to the stabilization problem under L1-gain control scheme is formulated through tractable LP conditions. Numer- ical examples and simulations are given to illustrate the effectiveness of the proposed control method presented in this chapter. Main content of this chapter is written based on paper [P2] in the List of publications. 3.1. Problem statement Consider the following LTI system with multiple delays x˙(t) = A0x(t) + m∑ k=1 Akx(t− hk) +Bww(t), t ≥ 0, (3.1a) z(t) = C0x(t) + m∑ k=1 Ckx(t− τk) +Dww(t), t ≥ 0, (3.1b) x(t) = φ(t), t ∈ [−d, 0], (3.1c) where x(t) ∈ Rn is the state vector, z(t) ∈ Rnz and w(t) ∈ Rnw are the regulated output and exogenous disturbance input vectors, respectively. A0, Ak, Bw, C0, Ck and Dw (k = 1, 2, . . . , m) are given real matrices with appropriate dimensions. hk and τk are known scalars involving time delays and d = max1≤k≤m{hk, τk}, φ ∈ C([−d, 0],Rn) 55 is the initial condition initializing the system state on interval [−d, 0]. To explicitly mention the initial condition, we will denote as x(t,φ) the corresponding solution of (3.1) with initial function φ ∈ C([−d, 0],Rn). Definition 3.1.1 ([32]). System (3.1) is said to be positive if its state and output tra- jectories x(t), z(t) are always nonnegative for any φ(t) ≽ 0 and w(t) ≽ 0. Proposition 3.1.1 ([12, 41]). System (3.1) is positive if and only if the following con- dition holds ⎧⎪⎪⎪⎨⎪⎪⎪⎩ A0 ∈Mn, S = [ A1 A2 · · · Am Bw ]
File đính kèm:
luan_an_bai_toan_dieu_khien_doi_voi_mot_so_lop_he_tuyen_tinh.pdf
Hong_Tomtat_uni.pdf
Hong_Abstract_uni.pdf
3-Thông tin kết luận mới_signed.pdf