Luận án Giải pháp học thích ứng trên nền tảng mạng học sâu ứng dụng nhận dạng đối tượng tham gia giao thông
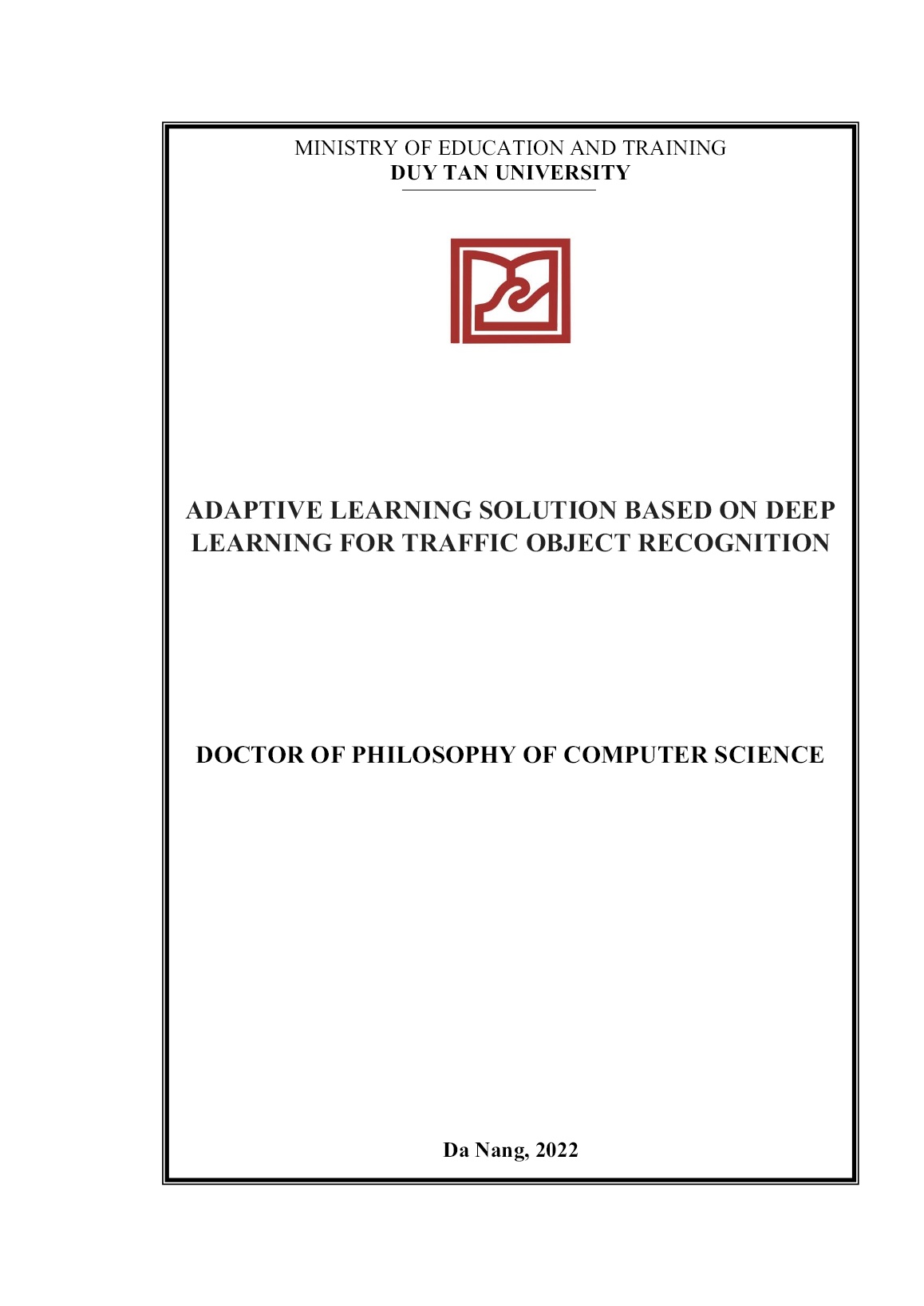
Trang 1
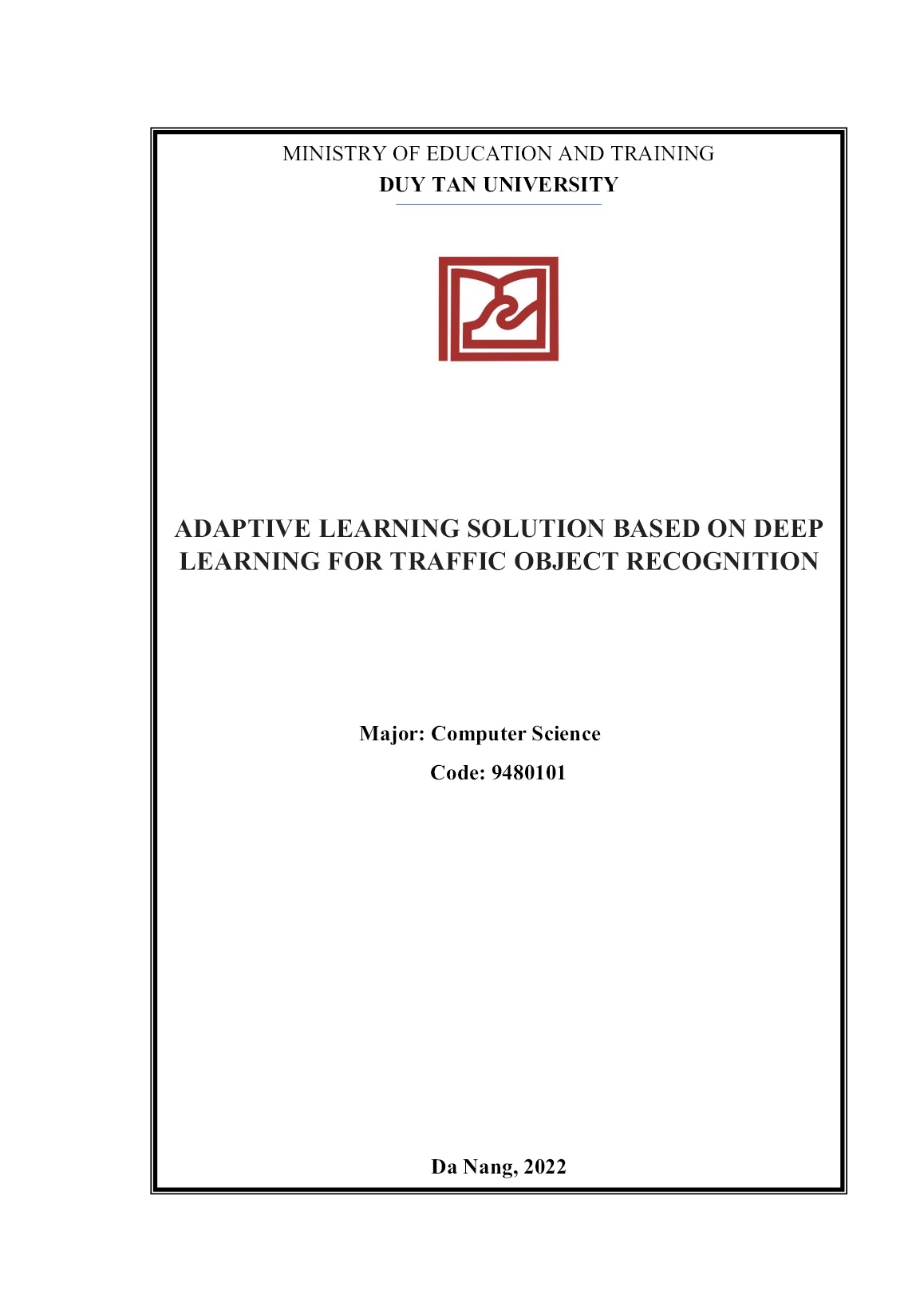
Trang 2
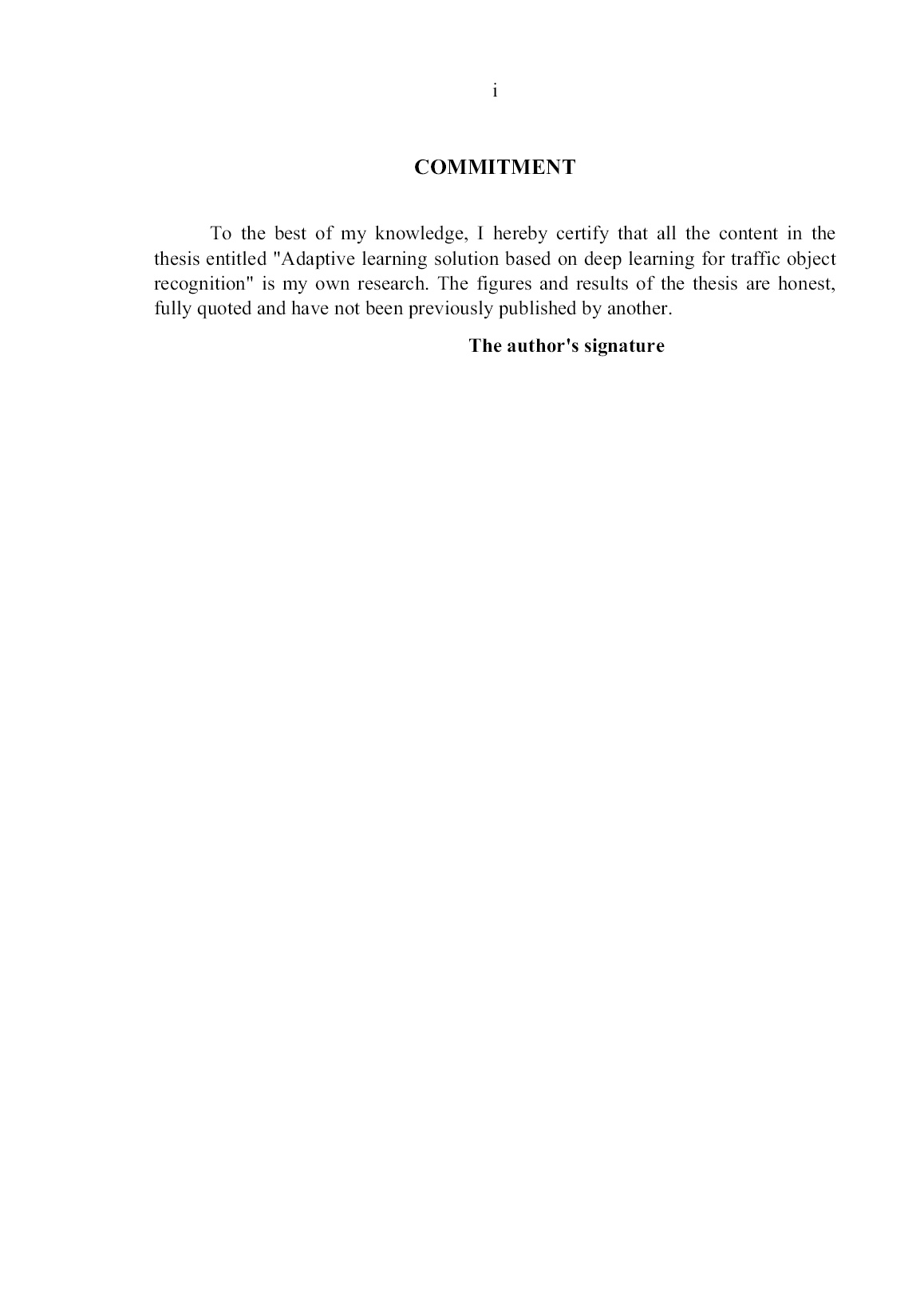
Trang 3
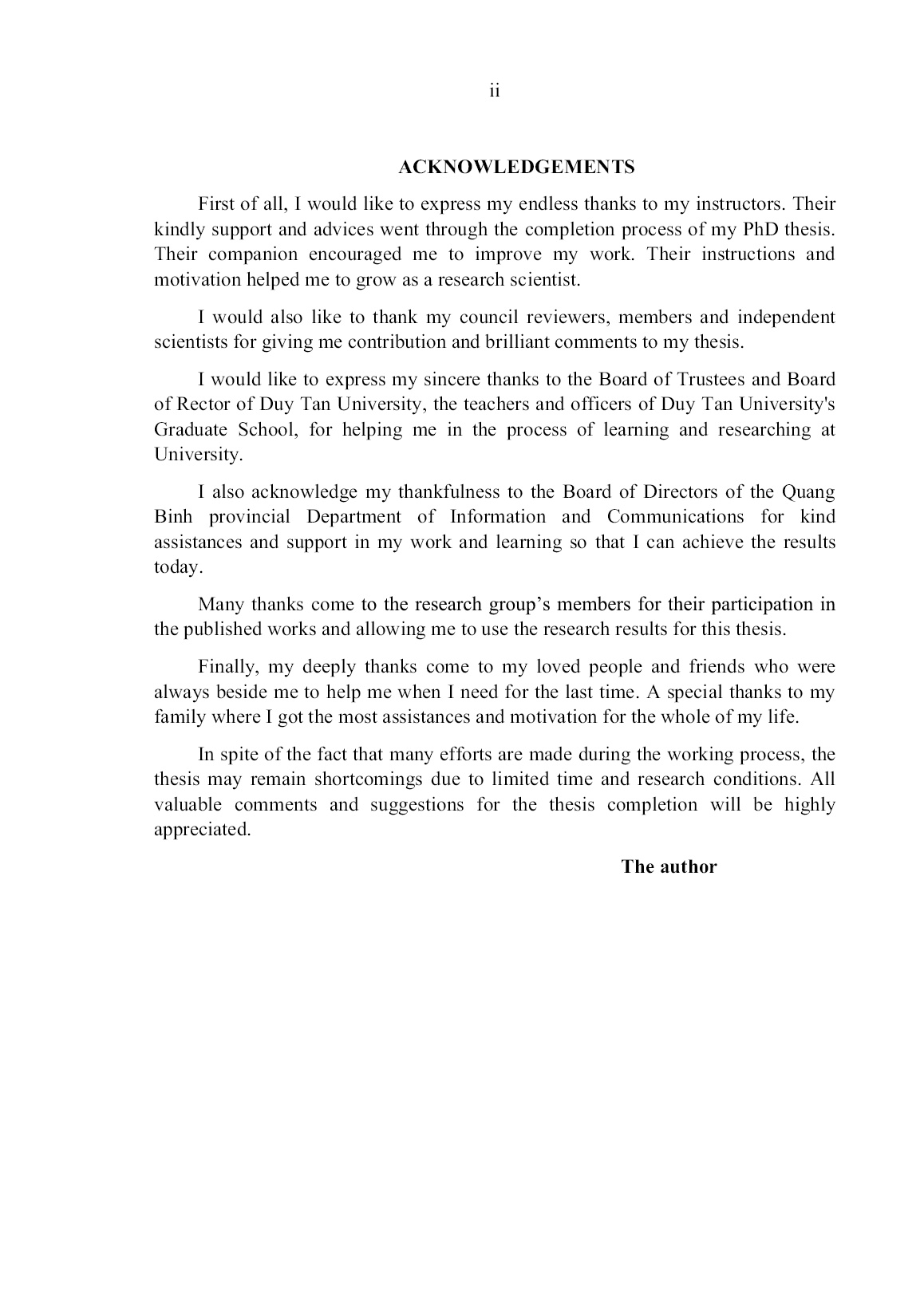
Trang 4
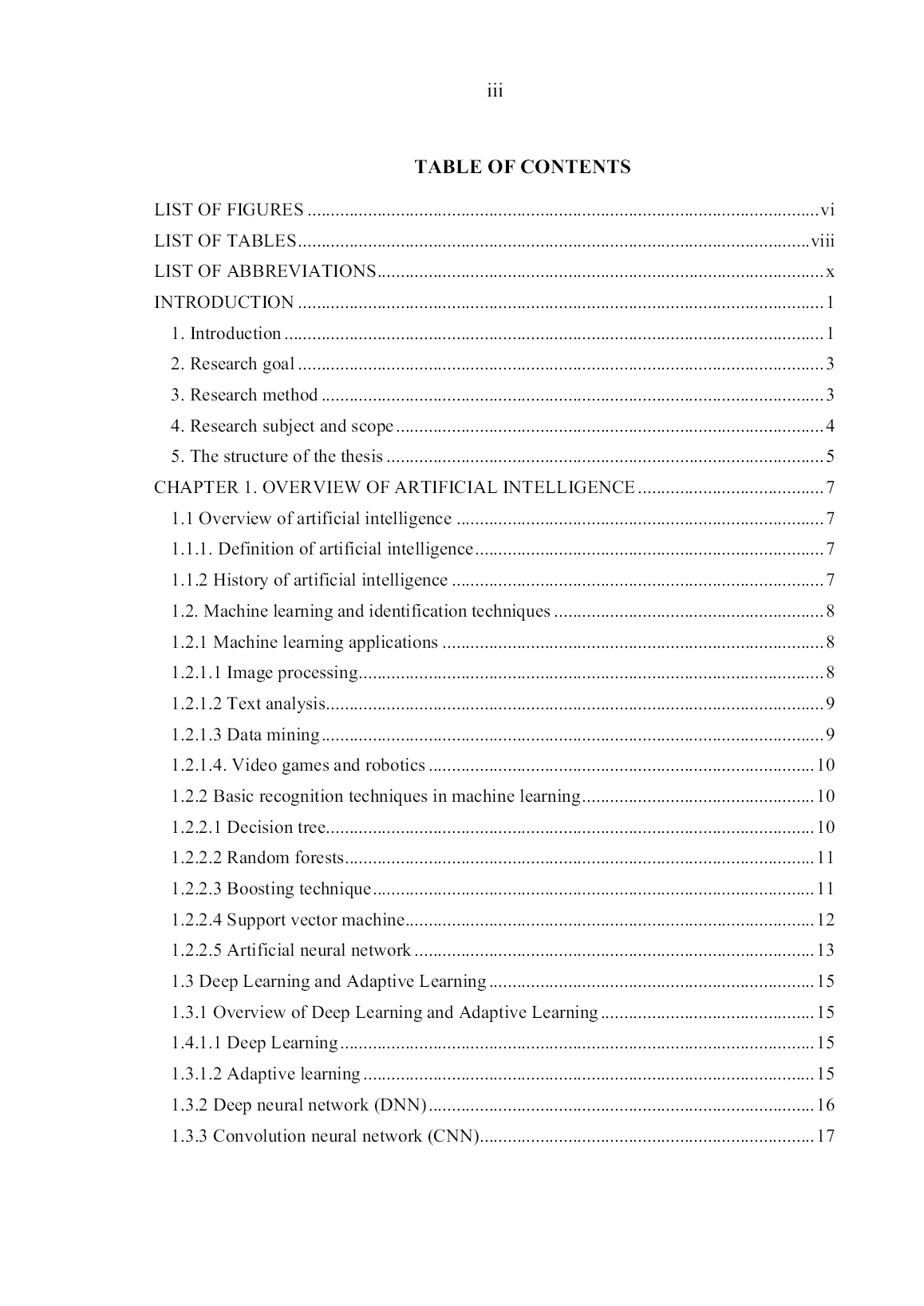
Trang 5
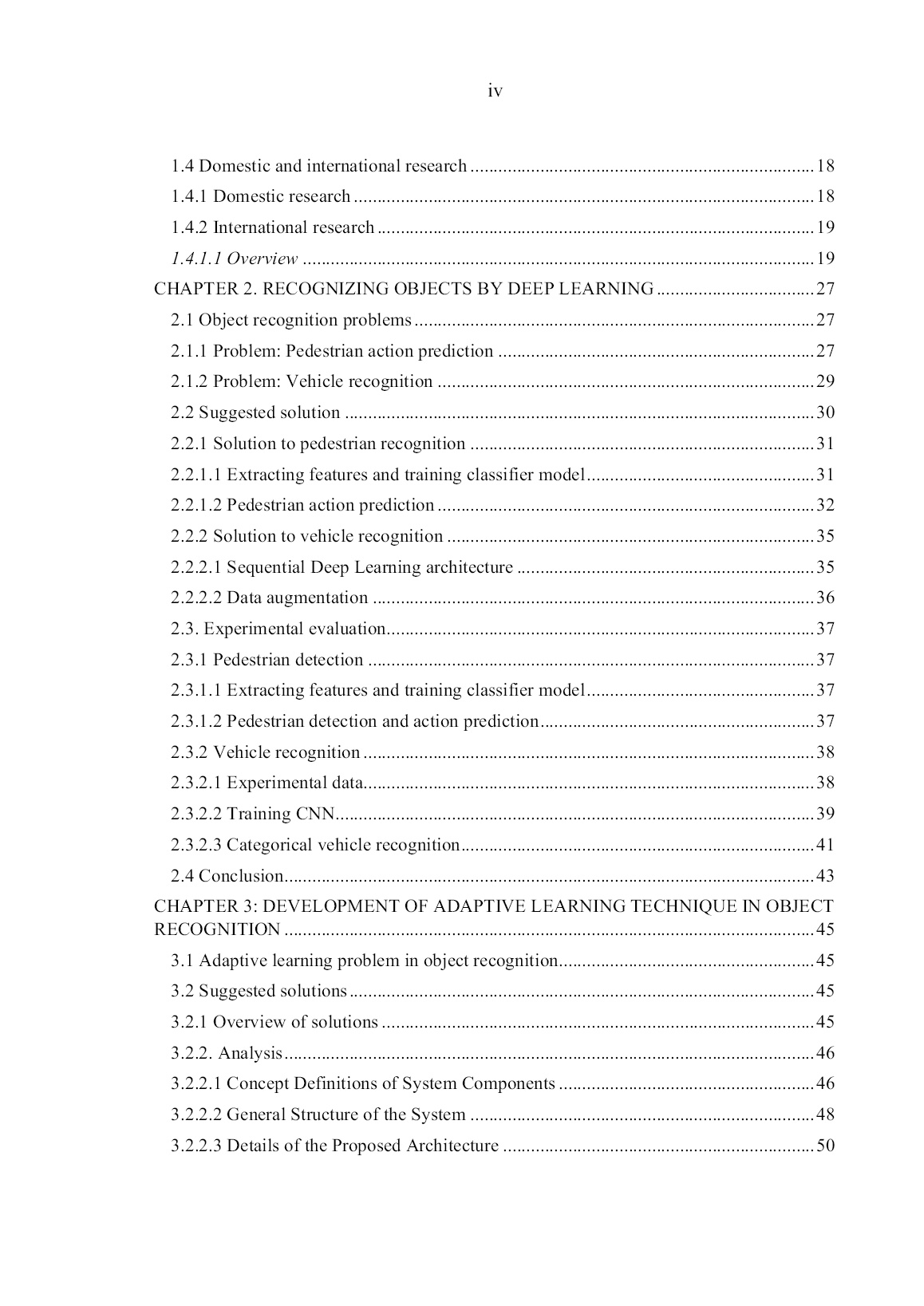
Trang 6
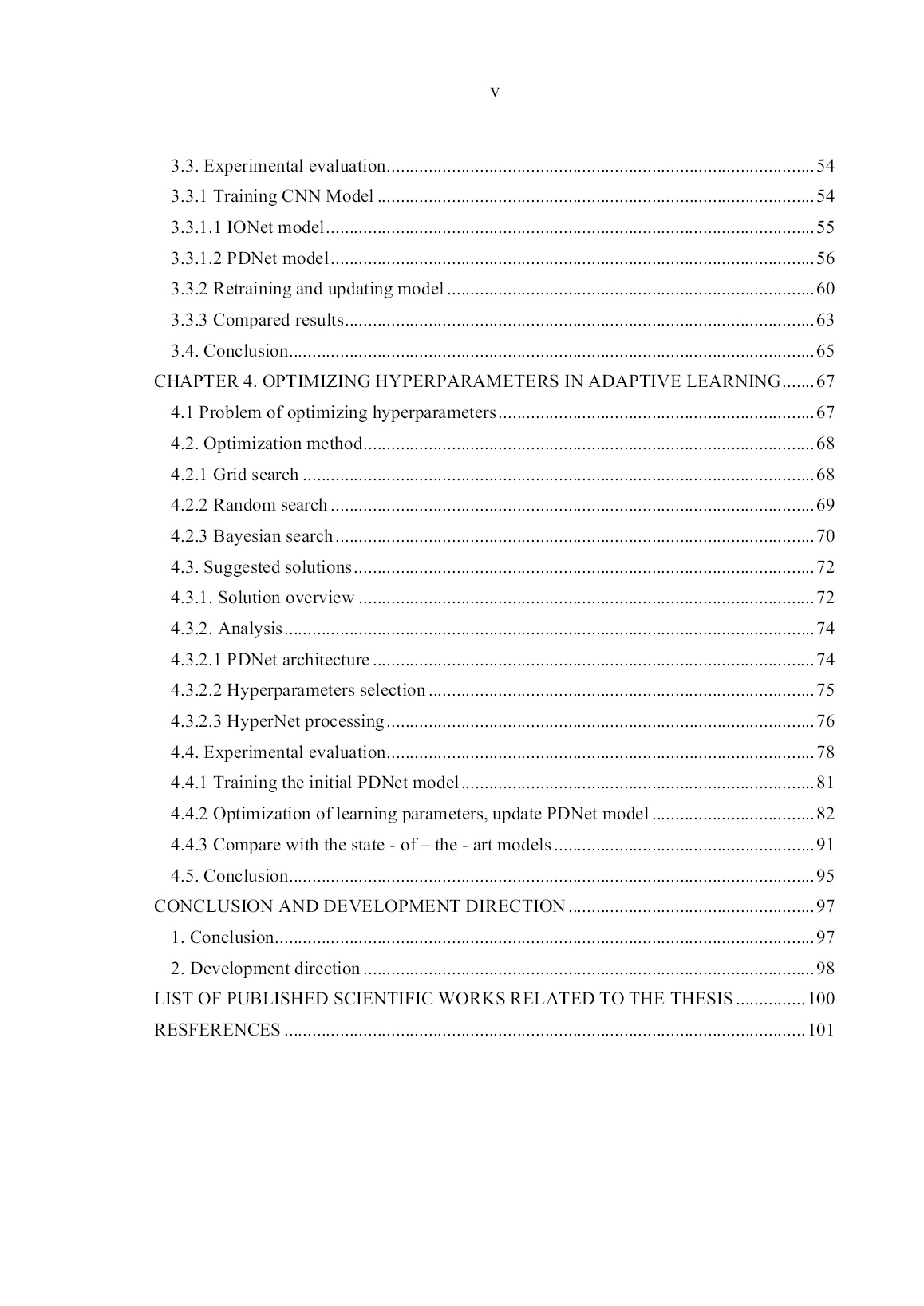
Trang 7
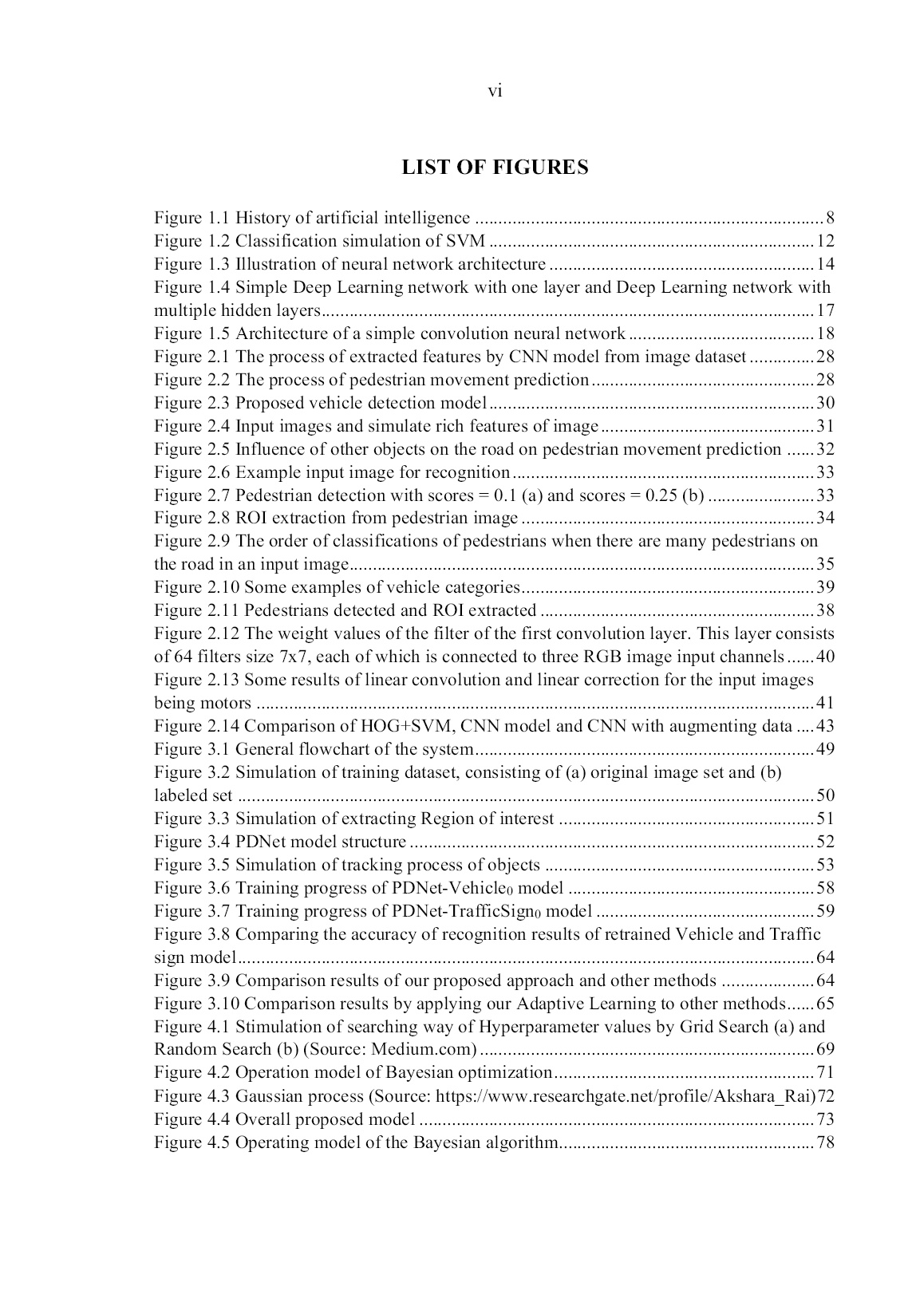
Trang 8
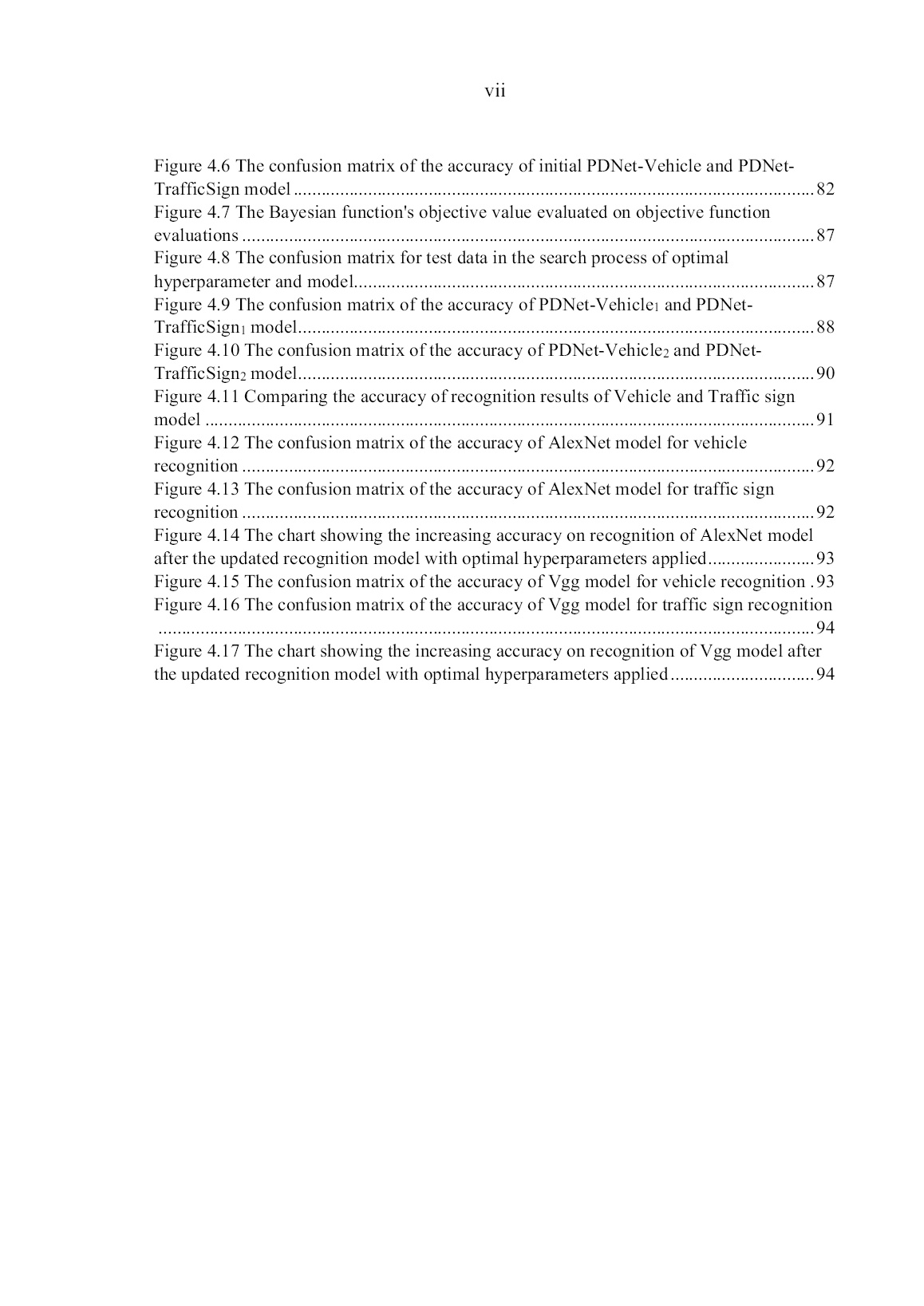
Trang 9
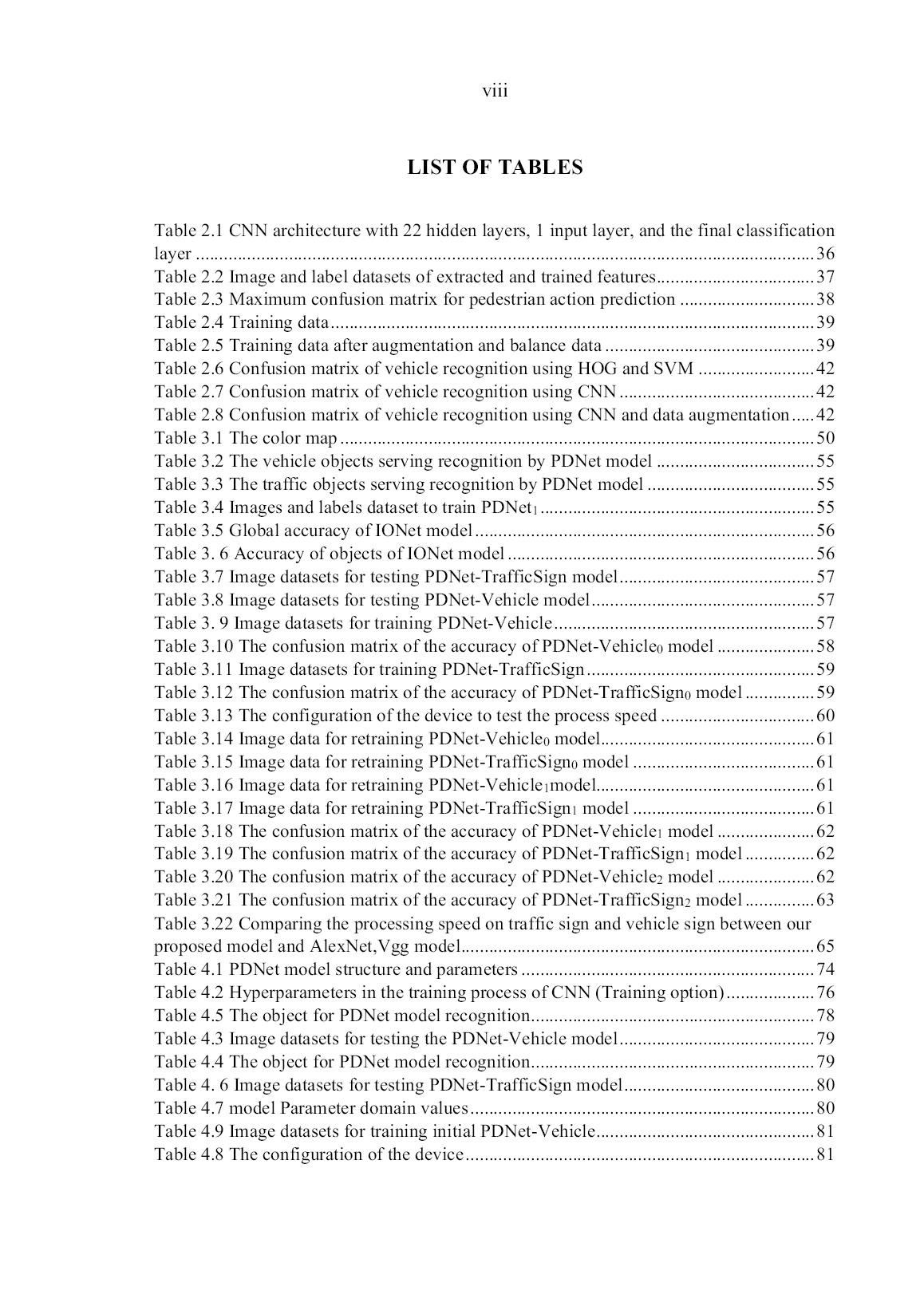
Trang 10
Tải về để xem bản đầy đủ
Bạn đang xem 10 trang mẫu của tài liệu "Luận án Giải pháp học thích ứng trên nền tảng mạng học sâu ứng dụng nhận dạng đối tượng tham gia giao thông", để tải tài liệu gốc về máy hãy click vào nút Download ở trên.
Tóm tắt nội dung tài liệu: Luận án Giải pháp học thích ứng trên nền tảng mạng học sâu ứng dụng nhận dạng đối tượng tham gia giao thông
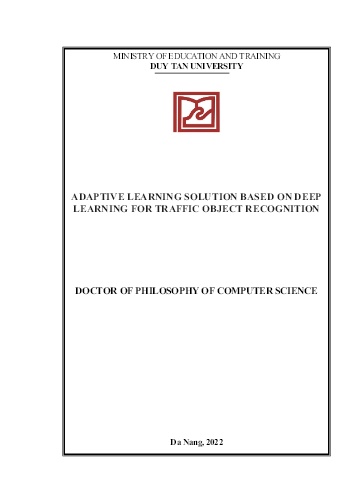
table frames) ( With each image, the CNN will extract the rich features, which are pedestrian postures, roadways, roadsides and positions of pedestrians on road, figure 2.5. The rich features extracted will be used for training SVM classifier model. a) Input image. b) Rich features simulation. Figure 2.4 Input images and simulate rich features of image 32 . In CNN model, many feature layers can be extracted such as convolution layer or full connected layer but the more advantageous layer is layer 19 (fc7 – 4096 fully connected layer) – the one right before the classification layer. Literally, in cases of object recognition such as animals, things and vehicles, the rate of recognizing object is higher (90% to 100%). In case of predicting the action of pedestrians, the features of input images focus on not only a specific object but also others such as vehicles, buildings, trees, and things around roadsides as shown in Figure 2.5. Figure 2.5 Influence of other objects on the road on pedestrian movement prediction In this regard, in term of accuracy, ACF algorithm is used to detect pedestrians before extracting ROI, classifying and predicting the action of pedestrians. 2.2.1.2 Pedestrian action prediction Pedestrian detection by ACF. ACF classification model, specified as 'inria- 100x41' or 'caltech-50x21', is person detection. The 'inria-100x41' model was trained using the INRIA Person data set. The 'caltech-50x21' model was trained using the Caltech Pedestrian dataset. The ‘inria-100x41’ model (default) is proposed in ACF. In ACF algorithm, detection scores value - confidence value - return an M-by-1 vector of classification scores in the range of [0..1]. When a pedestrian is detected, a bounding box will appear. The scores on top of bounding box are confidence value (by percentage). Larger score values indicate higher accuracy in the detection. In some complex images, the ACF algorithm sometimes 33 recognizes errors. During the real-time experimental process, the score value 0.25 is proposed to avoid error-recognizing cases. For example, if the score value is 0.1, the result will not be accurate in some cases (Figure 2.7 (a)) and if the score value is 0.25, the result will be of higher accuracy (Figure 2.7 (b)). Figure 2.6 Example input image for recognition a) b) Figure 2.7 Pedestrian detection with scores = 0.1 (a) and scores = 0.25 (b) In particular, when the AV moves on the roads, there are some cases in which so many pedestrians appear in one frame of the video. Therefore, to ensure the accuracy, it is considered that a frame be extracted into many separate frames to be easily recognized in each case. The region extracted is called ROI (Figure 2.8). Also, in real-time, the image received from AV is in big size and contains a lot of irrelevant data. Hence, extracting ROI of image at a certain scale, which removes irrelevant objects around, is necessary for each pedestrian detected. Extracting ROI of image helps the CNN model extract the exact features and reduce the error rate in the process of action recognition and classification of the SVM. The size of ROI is 34 proposed as follows: Supposing that H and W are height and width of the rectangle covering pedestrian object; x and y are the coordinates of the top left of rectangle and Width and Height are the size of input image, the values x1, y1, W1, H1 describe the size of ROI which are defined as follow: ( ) ( ) 1 1.5 1 – 1 3 1 2 1 1 1 1 x x H y y W W W H H H W if W Width thenW Width if H Height then H Height = − = = + = + = = (1) In special cases, when x1, y1, W1, H1 are smaller than the edge value of the frame or bigger than the size of the input image, the values equal the edge values of the image. ( ) ( ) ( ) ( ) 1 0 1 0 1 0 1 0 1 1 1 – 1 1 1 1 – 1 if x then x if y then y if x W Width then x Width W if y H Height then y Height H = = + = + = (2) On the other hand, when ROI is out of image input size, the offset value of ROI on the opposite side is proposed in Figure 2.8. Figure 2.8 ROI extraction from pedestrian image . 35 Pedestrian movement prediction: After ROI is extracted into a single image, the features are extracted (by CNN model) to be classified (by SVM classifier model). The outputs are labeled according to values of prediction of pedestrian case (i.e., Pedestrian_crossing, Pedestrian_waiting, Pedestrian_walking). (i) Pedestrian_crossing: When a pedestrian is crossing or walking in the road of other vehicles. (ii) Pedestrian_waiting: When a pedestrian is standing on the roadside and waiting to cross. (iii) Pedestrian_walking: When a pedestrian is walking on the edges of the road. (1) (2) (3) Figure 2.9 The order of classifications of pedestrians when there are many pedestrians on the road in an input image 2.2.2 Solution to vehicle recognition 2.2.2.1 Sequential Deep Learning architecture Usually, available pre-trained network models can be used to re-train the vehicle recognition models. However, in our approach, reusing the trained model is inappropriate, as the size of the old models differs from the actual images obtained simultaneously. Besides, the training parameters do not support accuracy improvement. Some proposed models, such as AlexNet [53], GoogleNet [28],... are only effective for general recognition problems, not for this specific recognition problem. There are many different approaches to building a CNN model in vehicle recognition. In this study, we constructed a 24-layer CNN architecture, shown in Table 2.1, consisting of the input layer, convolution layer, rectified linear unit layer (ReLU), cross-normalization, max-pooling, and fully connected layer. The network model transforms the input image into a serial hierarchical descriptor. The neural aggregate input is the intensity values of the image applied to the CNN model. Input Pedestrian_crossing Pedestrian_waiting Pedestrian_walking 36 sample includes 128×128×3 images. In this model, filters at the first layer concern to three-color channels, namely R-G-B. Filters operate independently and jointly among hidden layers, involving three channels of the input image. The final layer handling the feature vector will be extracted into the classification layer. A convolutional layer implements a combination of mapped input images with a filter size nx× ny. Table 2.1 CNN architecture with 22 hidden layers, 1 input layer, and the final classification layer TT Layer type Parameter 1 Image Input image size 128x128x3 2 Convolution 64 7x7x3 convolutions with stride [1 1] 3 ReLU ReLU 4 Normalization Cross channel normalization 5 Max Pooling 3x3 max pooling with stride [1 1] 6 Convolution 64 7x7x64 convolutions with stride [1 1] 7 ReLU ReLU 8 Max Pooling 2x2 max pooling with stride [1 1] 9 Convolution 64 7x7x64 convolutions with stride [1 1] 10 ReLU ReLU 11 Normalization Cross channel normalization 12 Max Pooling 2x2 max pooling with stride [1 1] 13 Convolution 64 7x7x64 convolutions with stride [1 1] 14 ReLU ReLU 15 Max Pooling 2x2 max pooling with stride [1 1] 16 Convolution 64 7x7x64 convolutions with stride [1 1] 17 ReLU ReLU 18 Normalization Cross channel normalization 19 Max Pooling 2x2 max pooling with stride [1 1] 20 Fully Connected 1024 fully connected layer 21 ReLU ReLU 22 Fully Connected 4 fully connected layer 23 Softmax softmax 24 Classification Output crossentropyex with 4 other classes 2.2.2.2 Data augmentation The training data set classified during the collection is shown in Figure 2.10. 37 In order to improve the accuracy of vehicle recognition, we propose to augment data about 10 times. Images are rotated [-50, 50], flipped or added noise, yet no changes will be made to the image quality during training. The training data set after augmentation is shown in Table 2.5. 2.3. Experimental evaluation 2.3.1 Pedestrian detection 2.3.1.1 Extracting features and training classifier model The experiment is carried out with about 3,000 images being extracted by CNN model. There features are used for training of SVM classifier model. Table 2.2 shows the image and label datasets of extracted and trained features. Table 2.2 Image and label datasets of extracted and trained features Class Number Label Pedestrian crossing 1,000 Pedestrian_crossing Pedestrian waiting 1,000 Pedestrian_waiting Pedestrian walking 1,000 Pedestrian_walking 90% of images from each set is used for the training data and the rest 10% is used for the data validation. 2.3.1.2 Pedestrian detection and action prediction With the input images (i.e., Figure 2.6), after using pedestrian detection ACF algorithm, the output is executed as in Figure 2.11. In case of the input images with many pedestrians in a frame, we extract ROI into a single image for action prediction by SVM classifier as shown in Figure 2.11. Each image in Figure 2.11 will be extracted features; finally, the system will rely on the SVM classification model to conduct action prediction of pedestrian and issue appropriate alerts for AV accordingly in Figure 2.9. 38 Figure 2.10 Pedestrians detected and ROI extracted The maximum results of rate-recognition after training and comparing with dataset in Table 2.2 are as follow: Table 2.3 Maximum confusion matrix for pedestrian action prediction Pedestrian crossing Pedestrian waiting Pedestrian walking Pedestrian crossing 0.9796 0.0204 0 Pedestrian waiting 0.0612 0.9286 0.0102 Pedestrian walking 0.0102 0.0408 0.9490 The result of experiment in real-time video on the road gives minimum accuracy rate of 82%, maximum of 97% and the speed for processing reaching 0.6 second per pedestrian detected. They are promising results for potential self-driving. 2.3.2 Vehicle recognition 2.3.2.1 Experimental data We have conducted experiments on a real database of vehicles including motors, cars, coaches, trucks taken from actual traffic situations. Camera systems typically receive signals in front of or behind the vehicles in traffic. This dataset is 39 collected from different practical contexts on different traffic routes. The training dataset is divided into 4 different vehicle classes, including motors, cars, coaches, trucks simulated in Figure 2.10, with 8,558 vehicle images. The dataset was actually collected in Nha Trang city, Khanh Hoa province, Vietnam. Dataset is partitioned into 60% for training and the remaining 40% for evaluation as shown in Table 2.4. (a) Motor (b) Car (c) Coach (d) Truck Figure 2.11 Some examples of vehicle categories Table 2.4 Training data Categories Number of samples Sample size Overall Train Evaluation Motor 2673 1604 1069 128x128 Car 2808 1685 1123 128x128 Coach 1640 984 656 128x128 Truck 1437 862 575 128x128 Table 2.5 Training data after augmentation and balance data Categories Number of samples Motor 16040 Car 16850 Coach 17712 Truck 17240 2.3.2.2 Training CNN Result obtained after CNN model training is shown as follows: 40 (i) Filter parameters: The first convolution layer uses 64 filters, whose filter's weight is shown in Figure 2.12: Figure 2.12 The weight values of the filter of the first convolution layer. This layer consists of 64 filters size 7x7, each of which is connected to three RGB image input channels (ii) Convolution result: The sample images fed into the network through a convolution filter and the obtained data show components distinct from the original RGB image with various feature result, creating a variety of vehicle features. The output value of the convolution set contains a negative value, which should be normalized by linear adjustment. The output of some layers is shown below, with the input pattern of the motor sample. (a) The output of 64 convolutions at the first convolution layer 41 (b) The linear correction value after the first convolution layer (c) The output of 64 samples at the second Convolution layer Figure 2.13 Some results of linear convolution and linear correction for the input images being motors 2.3.2.3 Categorical vehicle recognition Based on the experiment, three different methods have been evaluated on the same set of sample data as shown in Table 2.4. Methods include: (i) Traditional methods of HOG and SVM; (ii) CNN network; (iii) CNN network in combination with data augmentation. 42 The accuracy of the HOG and SVM method on the sample data set was 89.31%. Details of the sample size for each type and recognition result are shown in Table 2.6. Table 2.6 Confusion matrix of vehicle recognition using HOG and SVM Motor Car Coach Truck 1069 1123 656 575 #Num Per(%) #Num Per(%) #Num Per(%) #Num Per(%) Motor 1029 97.26 16 1.53 15 1.87 9 1.75 Car 25 2.36 989 94.37 77 9.59 32 6.23 Coach 1 0.09 23 2.19 599 74.60 33 6.42 Truck 3 0.28 20 1.91 112 13.95 440 85.60 The evaluated accuracy of the CNN method based on original data was achieved 90.10% on average, as shown in Table 2.7. Table 2.7 Confusion matrix of vehicle recognition using CNN Motor Car Coach Truck 1069 1123 656 575 #Num Per(%) #Num Per(%) #Num Per(%) #Num Per(%) Motor 1026 95.98 38 3.38 1 0.15 5 0.87 Car 32 2.99 953 84.86 17 2.59 24 4.17 Coach 6 0.56 104 9.26 617 94.05 58 10.09 Truck 5 0.47 28 2.49 21 3.20 488 84.87 The evaluated accuracy of the CNN method based on data augmentation was achieved 95.59% on average, as shown in Table 2.8. Table 2.8 Confusion matrix of vehicle recognition using CNN and data augmentation Motor Car Coach Truck 1069 1123 656 575 #Num Per(%) #Num Per(%) #Num Per(%) #Num Per(%) Motor 1060 99.16 11 0.98 0 0 1 0.17 Car 5 0.47 1057 94.12 8 1.22 13 2.26 Coach 0 0 41 3.65 645 98.32 51 8.87 Truck 4 0.37 14 1.25 3 0.46 510 88.70 43 In this study, we also evaluated the proposed CNN model to another traditional approach based on HOG feature descriptor and SVM classifier. Results of the comparison are shown in Figure 2.14. Figure 2.14 Comparison of HOG+SVM, CNN model and CNN with augmenting data 2.4 Conclusion Artificial intelligence with the development of machine learning, especially recent Deep Learning network, has brought great improvements in computer systems. Study content in Chapter 2 demonstrates the ability to recognize objects of CNN models and intelligence of CNN models in specific cases. Although the study was conducted in a small recognition area, the content clearly demonstrates basic techniques of Deep Learning in recognizing objects and the potential of application. However, a limit of artificial intelligence is the lack of self-study, self-update and self-thinking capabilities. Artificial intelligence would become perfect if learning and data training do not need the interference of humans. Therefore, Chapter 3 aims to build an Adaptive Learning to help autonomous systems in self-study, self-update and self-thinking to narrow the gap between artificial and human intelligence. In the 44 Chapter 2, the author mentions the two research works which are papers PP 1.1, PP 1.2, PP 1.3. 45 CHAPTER 3: DEVELOPMENT OF ADAPTIVE LEARNING TECHNIQUE IN OBJECT RECOGNITION In this Chapter, basing on the research results stated in Chapter 2, the Adaptive Learning solution of self-driving vehicle system data is continuously proposed. The proposed model is capable of self-learning and self-intelligence without any human intervention 3.1 Adaptive learning problem in object recognition Nowadays, object recognition techniques have achieved high accuracy due to the advent of advanced technologies, such as the deep convolutional neural network. With the growing support of computer hardware, the CNN models have increasingly complex structure, more layers, and a large amount of training data. These systems are capable of identifying most object classes with high accuracy. However, the models just well recognize objects in the case they are a high similarity to the trained data. Meanwhile, the change of status or appearance of objects existing in practice is considerable variety and the image obtaining process of devices is affected by environmental conditions, such as brightness, rain, fog, vibration by movement, etc. Thus, the training dataset, large as it is, cannot cover almost all status of objects in practice. Additionally, training on too large data sets leads to impossible task due to limited computer resources and consuming time. To deal with these problems, proposed an approach solution, which is adaptive for automatically upgrading the recognition model with expected to reach higher accuracy. 3.2 Suggested solutions 3.2.1 Overview of solutions In this chapter, a solution will be suggested based on Adaptive Learning by CNN models. In this suggested method, the recognition model will automatically update by directly collecting data in the normal operation of an ADAS, training, comparing the accuracy and updating the model. The updating mission will focus on datasets that are different from those in previous training. The solution aims to 46 update the old model so that it would be more adaptive and accurate. In the Adaptive Learning method, recognition systems can learn and add information by themselves without the help of experts in data labeling. Especially, thank to the increasingly developed online storage technology, development of infrastructure and data transmission solutions on new platforms available (5G, Cloud data, etc.), the problems of the proposed model are expected to be handled by storage and updating of online data. Suggested solutions include five main stages: (1) Object detection with low reliability (2) Object tracking in n images in following processes to identify if they are objects of interest. (3) In case recognized objects with high reliability: label Positive for datasets recognized with low reliability in previous processes. In case recognized objects are not of interest, label Negative for all objected tracked in previous images. (4) Establishing a training dataset based on the collective combination of training dataset and new dataset. (5) Retraining and re-updating model if the new version has higher accuracy than the old one. Trials were conducted to compare suggested model PDNet with modern models such as AlexNet and Vgg. Results showed that the suggested model have higher accuracy than a model that is self-taught over time. Further, the suggested Adaptive Learning model can be applied with conventional recognition models such as AlexNet and Vgg to improve their accuracy. 3.2.2. Analysis 3.2.2.1 Concept Definitions of System Components Before going into detail the block functions of the system, some concepts are classified and defined as follows: (1) Adaptive learning The self-learning, self-adaptability of a Deep Learning model. The adaptive process supports to automatically improve the ability to recognize objects of the system without the need of manually data complementation and expert support. 47 (2) Interest objects (IO) The object of interest to detect and recognize; for example, traffic signs, vehicles, etc. (3) Confidence scores A measure of reliability when an object is detected as IO. The confidence score of object O is denoted as Conf(O). ConfidenceH is a highly confident threshold. (4) Confident tracking The process of object tracking when an object is detected as an IO. (5) Lost object (LO) Objects initially recognized as low confi
File đính kèm:
giai_phap_hoc_thich_ung_tren_nen_tang_mang_hoc_sau_ung_dung.pdf
5. Thong tin_Luan An_tieng Viet.pdf
4. Thong tin_Luan An_tieng Anh.pdf
3. Tom Tat Luan An_tieng Viet.pdf
2. Tom Tat Luan An_tieng Anh.pdf